Managing Big Data: Moving analysis back to the edge
July 20, 2016
Blog
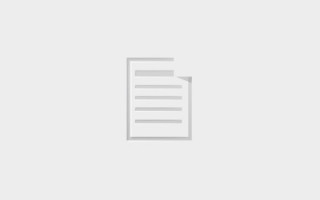
With all the gadgets we use each day, it's easy to lose sight of the fact that many of these devices have processing power equal to the old computer t...
With all the gadgets we use each day, it’s easy to lose sight of the fact that many of these devices have processing power equal to the old computer towers from just a decade ago. Add the Internet of Things (IoT) to the equation, and we’re generating a mind-blowing amount of data every second of every day.
According to Cisco, the world generated about two exabytes of data altogether in the past 2,000 years. Now we generate that amount of data daily.
The IoT relies on data — and massive amounts of it — to fuel connected devices and give us drastic advances in monitoring and automating our environments. With the information that the IoT provides through these devices, businesses and industries can know sooner and with more accuracy how the business is being affected by external factors. For example, in an industrial setting, IoT sensors are used to monitor and regulate the humidity of a manufacturing facility when dealing with a sensitive material. Data collected by a sensor triggers various action scenarios by the device.
Big Data also is being leveraged by businesses to better understand their customers and drive efficiencies. For example, the use of item-level RFID tags by retailers raises inventory accuracy from an average of 63 percent to 95 to 99 percent, according to the RFID Lab at Auburn University. Item-level RFID also can provide real-time data that can fuel more integrated shopping experiences by helping merchants track inventory status, purchase data, item popularity, and shopper movement.
With so much data being generated so quickly, data management becomes a challenge. Questions arise about the value of all the data, and how to best sort through it effectively so the data becomes actionable.
One solution is to develop more advanced network technology at the local level. With the cloud being a big source for the analysis of IoT data, moving the process back to the device itself could drive efficiencies. This process is called moving toward the edge, or more simply, edge IoT analytics.
Moving toward the edge is a simple idea: the sort of computations and data sorting that used to require a server can now be accomplished on the connected device itself. Moving analytics closer to the edge provides a number of benefits. Chief among those is cost savings. Bandwidth costs money, and the growing amount of data that will be sent via sensors and other devices will continue to grow. Edge analytics reduces the amount of back-and-forth needed to process the data.
Products that can monitor and synthesize information locally also are preferable because they naturally will have fewer latency issues. They can act quicker and with a higher data reflex.
As businesses and institutions look to IoT to become more competitive, the issue of how they process the vast amount of data these devices generate will be a serious consideration. The old method of relying on servers for the analytic and decision-making process will be replaced by more modern edge technologies that increase reliability and reduce costs.