The Perplexities of Predictive Maintenance: Synthesizing and Sourcing Adequate Amounts of Data
March 19, 2019
Story
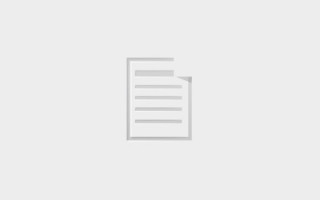
Here, we'll explore what happens when the challenge lies with the lack of data, the foundation of any predictive maintenance model.
In my first blog, I discussed data’s role in building a predictive maintenance model across five core development stages, and the challenges that arise when engineers are not educated on a systematic predictive maintenance workflow.
Here, we’ll explore what happens when the challenge lies with the lack of data—the foundation of any predictive maintenance model.
To build machine learning algorithms, which many predictive maintenance systems rely on, there must be enough data to create an accurate model. This data usually originates from sensors on machinery, but companies can run into issues when data collection is not an option, when using new sensors, or when data readings are incorrectly logged, and information is limited.
Each of these challenges is solvable. Below we discuss three common data accumulation scenarios as well as techniques and strategies for overcoming hurdles associated with each one.
Scenario 1: Ground Zero
In this scenario, your department does not collect enough data to train a predictive maintenance model, and you are unsure what additional data can be sourced and from where. Consider other internal departments that collect data and might be able to supplement your existing data. Sourcing within your organization might be enough to meet your needs.
Suppliers and customers also have the potential to supplement data, depending on the size of the business and where it lies in the supply chain. Explore existing agreements and determine whether a collaboration can be fostered. Offering to prolong the health and efficiency of equipment components is just one example of a benefit that would be appreciated across businesses. While this won’t always be possible, the volume of data that could be acquired merits consideration.
Scenario 2: Feast or Famine
Here, a department has the tools to capture an adequate amount of data, but the system cannot collect it until a fault occurs. Or worse, the system can only collect event codes and time stamps, meaning sensors are not collecting data values crucial for developing models that can predict those failures.
Companies can increase the efficiency at which they capture data by changing data logging options on the internal system, perhaps on a test fleet if production data is not available. It may even be possible to collect and transmit sensor data by reconfiguring existing embedded devices, though external data loggers may be needed when getting started (Figure 1).
Scenario 3: Simulation Software
In certain scenarios, simulation tools can play a strong role in helping teams generate test data and combine it with available sensor data to build and validate predictive maintenance algorithms. Data generated by simulation tools should be compared to measured data, to make sure the simulation is well-calibrated. For example, a DC server motor model could be built and then calibrated using real-world sensor data.
Analyze Early and Strategically
The lack of data can create significant issues for your predictive maintenance system. Fortunately, there are several solutions that engineering teams can employ to source, combine, and even generate their own data. Regardless of your specific data needs, all businesses considering data for predictive maintenance should begin analyzing early and strategically. Once you’ve come to understand the data features that are most important for your goals, you can make informed decisions about which data need to be kept and which do not.
In my third and final blog, I will continue to explore challenges associated with predictive maintenance data—specifically, root cause analysis. I will break down the benefits of this problem-solving method and share common challenges that arise when either there is a lack of failure data or an inexperienced engineering team must come to terms with the concept.