Filling the data scientist gap, part 1: Turning engineers into data scientists
February 06, 2018
Blog
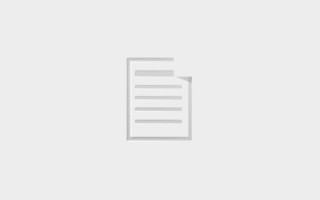
There?s a shortage of data scientists and companies are struggling to fill the void ? but they may find success by focusing on candidates with domain expertise.
There’s a shortage of data scientists and companies are struggling to fill this void – this isn’t new information in the data science space. Companies are looking for data scientists who have computer science skills, knowledge of statistics, and domain expertise relevant to their specific business problems. These types of candidates are proving elusive, but companies may find success by focusing on the latter.
This third skill – domain expertise about the business – is often overlooked. Domain expertise is required to make judgement calls during the development of an analytic model. It enables one to distinguish between correlation and causation, between signal and noise, between an anomaly worth further investigation and “oh yeah, that happens sometimes”. Domain knowledge is hard to teach: It requires on-the-job experience, mentorship, and time to develop. This type of expertise is often found in engineering and research departments that have built cultures around understanding the products they design and build. These teams are intimately familiar with the systems they work on. They often use statistical methods and technical computing tools as part of their design processes, making the jump to the machine learning algorithms and big data tools of the data analytics world manageable.
With data science emerging across industries as an important differentiator, these engineers with domain knowledge need flexible and scalable environments that put the tools of the data scientist at their fingertips. Depending on the problem, they might need traditional analysis techniques such as statistics and optimization, data-specific techniques such as signal processing and image processing, or newer capabilities such as machine learning algorithms. The cost of learning a new tool for each technique would be high, so having these tools together in one environment becomes very important.
Staying current and flexible
A natural question to ask is, how can newer techniques like machine learning be made accessible to engineers with domain expertise? Let’s dive a little deeper into the technology to come up with an approach.
The goal of machine learning is to identify the underlying trends and structure in data by fitting a statistical model to that data. When working with a new dataset, it’s hard to know which model is going to work best; there are dozens of popular models to choose from (and thousands of less-popular choices). Trying and comparing several different model types can be very time-consuming using "bleeding edge" machine learning algorithms. Each of these algorithms will have an interface that is specific to the algorithm and preferences of the researcher who developed it. Significant amounts of time will be required to try many different models and compare approaches.
One solution is an environment that makes it easy for engineers to try the most-trusted machine learning algorithms and that encourages best practices such as preventing over-fitting. For example, the process engineers at a large semiconductor manufacturing company were considering new ways to ensure alignment between the layers on a wafer. They came across machine learning as a possible way to predict overlay between layers but, as process engineers, they didn’t have experience with this newer technique. Working through different machine learning examples in MATLAB, they were able to identify a suitable machine learning algorithm, train it on historical data, and integrate it into a prototype overlay controller. The flexible MATLAB environment allowed these process engineers to apply their domain expertise to build a model that can identify systematic and random errors that might otherwise go undetected.
According to Gartner, engineers with the domain expertise “can bridge the gap between mainstream self-service analytics by business users and the advanced analytics techniques of data scientists. They are now able to perform sophisticated analysis that would previously have required more expertise, enabling them to deliver advanced analytics without having the skills that characterize data scientists.”
As technology continues to evolve, organizations must quickly ingest, analyze, verify, and visualize a tsunami of data to deliver timely insights to capitalize on business opportunities. Instead of spending time and money searching for those elusive data scientists, companies can stay competitive by enabling their engineers to do data science with a flexible tool environment like MATLAB that enables engineers and scientists to become data scientists – opening up access to the data for more people.