How today's AI technology will deliver tomorrow?s advancements
December 14, 2017
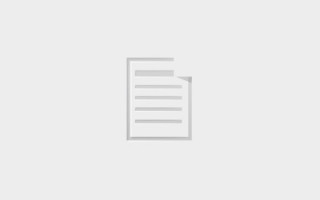
Today AI technology exists in many forms, in some cases operating autonomously, but more commonly as a human aide.
Research shows that consumers have concerns about how AI technology might impact their lives in the next five to ten years, which is understandable given that change normally promotes anxiety – but history shows us that society tends to benefit from technological advancement.
Although the concept of AI dates back to the 1950s, AI technology is still relatively young and comes at a time when many other kinds of innovation vie for our attention, such as new internet services, autonomous vehicles, and digital assistants. In reality, although it may not be obvious, all of these technologies will be increasingly enabled by developments in AI. To some extent, they already are.
Today AI technology exists in many forms, in some cases operating autonomously, but more commonly as a human aide. These point solutions may be connected, but are unlikely to be operating collaboratively – at least not yet. As such, there is no single entity that has the ability to control every aspect of our lives or hatch a plan for world domination.
Learning vs. teaching
Many forms of AI are being developed. One of the highest profile is machine learning, which effectively allows a machine to alter its own processing and reactions. Learning algorithms underpin many of the examples of AI technology now being used in tasks that require this kind of acquired, experienced-based intelligence or learning.
Conversely, expert systems are examples of how AI is used in a more controlled application. The developer/manufacturer includes all the information a machine/device needs to carry out a specific task or tasks, in an environment that is unlikely to present unforeseeable challenges.
In the real world, unforeseeable challenges are everywhere. For this reason, systems that fulfill the popular image of AI, such as self-driving cars, will rely on algorithms that are able to learn. The question then becomes, how much knowledge must such a system have before it becomes reliably useful?
Fortunately, the learning process is transferable: what one machine learns can be used by another. This level of collaboration is necessary to accelerate the pace of innovation in AI systems. Another trend that will accelerate the integration of AI technology into consumers' lives is natural language processing, or the ability to talk with an AI naturally as if it were another person.
This raises an important point, and one that has existed since the birth of artificial intelligence: do we really expect AI to be indistinguishable from a human? The Turing Test is often cited whenever this topic is raised, but it presupposes that the intention is to present an AI as human. Research suggests that only a minority of consumers expects AIs to physically appear as human. The majority expect AI technology to be embedded in a device and thus be largely invisible. This latter scenario is not only more likely, but also much more feasible. Here’s an interesting video that lays this out.
Cloud or edge?
If there is a single trait that betrays an AI system attempting to present itself as human, it is probably latency. The time it takes for a machine to process information and formulate a response is perceptible to a human observer. This is key. When you speak to a device, you don't expect it to be offline and unresponsive. This is one reason AI is moving to the edge, where devices increasingly can handle some portion of AI processing.
While latency is always a key consideration, it’s sometimes a critical one. A vending machine that attempts to predict stock levels based on weather, time of day, or location can tolerate much longer latencies. An autonomous vehicle needs extremely low processing latencies.
Digital assistants currently use cloud computing for natural language processing, but as this develops, the algorithms could be transferred to the edge, allowing a faster response. The same principle applies to other forms of AI in devices that participate in larger, connected systems. As decision making moves closer to the edge, automation becomes faster and therefore applicable in more scenarios.
Consumer demand is also driving more compute to the edge. Recent research shows that half of the consumers would find fully autonomous vehicles that are proven to improve driver and passenger safety appealing, while even more like the idea of AI-controlled traffic signals that can improve traffic flow in busy periods. Both applications would require significant amounts of processing power for sensing and local computing capabilities.
At a higher level, AI-enabled devices will share critical data that would further enable decision making on a larger scale.
The ability to make decisions that aren’t predetermined will really differentiate systems that employ AI, particularly in applications where the environment, is unpredictable. As algorithms are optimized and processors become more powerful and optimized for running AI software, decision making at the edge is already opening a wider range of applications.
Conclusion
The potential for AI technology to improve society isn’t in doubt, and while this will inevitably be met with some resistance, its use is already widespread and continues to expand. In other words, the dystopian vision that we see and read in fiction is, in fact, fiction. Indeed, most humans are now asking, ‘What else can artificial intelligence do for me?’
To find out more about how consumers view the future potential of AI, check out these global survey results.