AI-Based Anomaly Detection Enhances Performance of Industrial Machinery
October 05, 2021
Blog
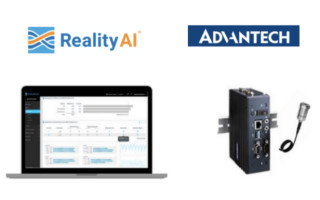
Apart from lengthy and expensive operational research, factory production can meet its optimal targets by thorough analysis and monitoring of the overall system. Additionally, production workflow is efficient when machinery works at its maximum efficiency without faulty intervals or anomalies.
Credits: Reality AI
Traditional methods of machine-life evaluation generally focus on diagnosis and lack the ability to predict faults or threats. Hence, the integration of AI brings offers a solution by introducing the concept of machine-level predictive analytics.
Generally, systems fail due to the deterioration of the components or any external force which disturbs the overall procedure. So, it can be interpreted that there has to be some anomaly from the normal behavior for a system to break down. Most of the time these anomalies occur gradually rather than abruptly, which can make it possible for the detection and prediction of that anomaly before it makes a major impact on the system.
Advantech Edge Node for Sensor Data Collection
Data collection plays a significant role in performing exploratory machine-level analytics followed by fitting the dataset with respect to AI models for anomaly detection. The physical features of the machinery need to be measured using a range of sensors for evaluating the condition and performance.
Credit: Author
Reality AI and Advantech have partnered on an Edge Node for evaluating the machinery for detecting anomalies and predicting the life expectancy of the running components. It is named as RealityCheck AD Edge Node based on Advantech’s EPC-S201 fanless embedded PC. Intel’s Celeron N3350 Dual-Core SoC constitutes the heart of the embedded PC and it features 8GB RAM and 64G SSD showing an extensive processing and storage capacity.
It comes with a wide range of sensor options for data collection from the machinery:
- Accelerometers from several different makers
- Current and temperature sensors for performance and heat evaluation
- Contact microphones for audio sensing of the machinery
The Edge node is flexible to deployment as it comes with options for wall mounting and rail mounting, which comply with the majority of the deployment possibilities in industries. Additionally, the device supports a wide range of connectivity including the support of Wi-Fi, ethernet, and cellular communication.
Anomaly Detection using Reality AI Software Tools
The sensor data needs to be cleaned and pre-processed before applying any AI algorithm. Reality AI software efficiently extracts the features from the data after thorough pre-processing and cleaning. It uses an algorithmic search with a wide range of feature spaces to derive a custom transform based on time and frequency. The software offers optimal results in the case of anomaly detection, signal classification, and life expectancy prediction.
Reality AI also comes with the functionality to adapt the weights of AI models depending on the use case. It can also create machine learning models and data visualization for better accuracy and understanding of the application. Additionally, it provides in-depth hardware analytics which helps in the solution design.
Credits: Reality AI
RealityCheck AD comes with a baseline normal strategy for optimizing the anomaly detections. The method evaluates the reference region in the feature space and the scores compare the observation with the baseline normal region, which is actually the reference region. Reality AI software predicts the correct feature space for baseline normal by classifying the compactly packed region. All the known anomalies are distant from the edges of the baseline, therefore, the distant points become the anomalies thus completing the detection process.
Credits: Datasheet
The flow of anomaly detection starts with the creation of the baseline region in the initial detection model. The real-time data collection is continuously updated in the dataset, at the same time the deployed detection model predicts anomalies in the system. This prediction is investigated and verified, in order to find out if the prediction is completely accurate. In the case of true predictions, the particular data point is labeled as an anomaly and updated in the dataset. Similarly, in case of false prediction, the data is labeled and updated accordingly.
Although the AI models are accurate, there might be a few anomalies that may go undetected due to noise or overfitting. But most of them are detectable in terms of equipment monitoring and end of line testing. With equipment monitoring being the foundational brick of machine-level analytics, the insights of failure can save a lot with respect to the overall production. Additionally, end of the line testing ensures quality and performance, making it significant from the safety perspective as well. RealityCheck AD Edge Node enhances production machinery to meet optimal targets.
Reality AI’s Anomaly Detection-based solution was showcased in the Sensors Converge event and will be featured at the Auto Tech Science Fair in October 2021. You can head over to the official page of RealityCheck AD for detailed information on the solution.