Not all Analog Computing Is Equal
July 01, 2021
Story
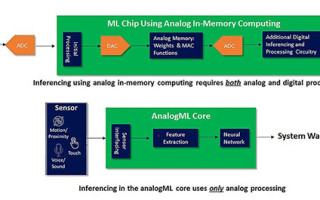
AnalogML enables system-level efficiency by operating completely within the analog domain.
With the explosion of always-listening devices in consumer, biomedical, and IoT/IIoT markets, it seems like everyone’s trying to use analog to save power in their designs. With a new crop of machine learning (ML) digital chips that use “analog in-memory computing” to reduce processor power, semiconductor suppliers are inventing new ways to take advantage of the inherent power and computational efficiencies of analog computing. So, what’s the hitch? The truth is that although these chips take advantage of the inherent benefits of analog circuitry to save power within the chip for neural network processing, they’re ultimately digital-processing chips that operate in the digital domain on digital data—which means that they only offer limited power-savings to the system. Fortunately, a new systems-level approach that uses analog computing more comprehensively—an analog machine learning (analogML) core—now enables far greater power efficiency at the system level.
While both analog in-memory computing and analogML are sometimes labeled “analog computing,” they’re in no way the same thing. What do designers need to know about the differences between analog in-memory computing and the analogML core, so they can create more power-efficient end devices?
Chip-level Efficiency from Analog-in-Memory Computing
Analog in-memory computing generally refers to using analog circuitry within the neural network of an otherwise digital machine learning processor in order to perform the multiply-accumulate (MAC) functions at lower power. But chips that leverage this approach are still clocked processors that operate within the standard digital processing paradigm, requiring the immediate digitization of all analog sensor data, relevant or not. In fact, a chip that uses analog in-memory computing actually requires three separate data conversions prior to determining the importance of the data. Sensor data are immediately converted to digital for initial processing (a digitize-first architecture), then they’re converted to analog within the chip for the MAC functions, and finally, they’re converted back to digital within the chip for the additional digital processing required for inferencing, classification, and other functions. So, plenty of data conversion, but not much actual analog processing.
While analog in-memory computing may reduce the power of an individual inferencing chip, it’s only using analog in a very limited way, so it only delivers equally limited power reductions to the overall system.
System-Level Efficiency with AnalogML
In contrast, the analogML core operates fully within the analog domain, with no clock required, and uses raw analog sensor data for inferencing and classification before digitizing any data. Integrated into an always-listening device, the analogML core determines the importance of data prior to spending any power on even a single data conversion. We call this “analyze first” because the analogML core keeps the digital system off unless relevant data are detected.
Compared to an ML chip that incorporates analog in-memory computing, the analogML core’s more streamlined approach to processing analog sensor data has a major impact on system-level efficiency. (See figure 1.)
Figure 1: Comparison of a digitize-first system architecture using an ML chip with analog in-memory computing (top block) to an analyze-first system architecture that uses an analogML core (bottom block)
For example, in a typical voice-first system, the analogML core is on 100% of the time, drawing as little as 10µA in always-listening mode to determine which data are important (analyze-first architecture), prior to spending any power on digitization. This keeps the rest of the system asleep until relevant data are detected. Compared to a more traditional ML chip that operates 100% of the time in the digital domain (digitize-first architecture), and draws a whopping 3000-4000µA, the analyze-first approach using analogML extends battery life by up to 10 times. That’s the difference between smart earbuds that last for days, instead of hours, or a voice-activated TV remote that lasts for years, instead of months, on a single battery charge.
What’s in the AnalogML Core?
Looking under the hood of the analogML core reveals the difference between analog in-memory computing, where analog computing is used just for the neural network, and the analogML core, which consists of multiple software-controlled analog processing blocks that we can enable, reconfigure, and tune for various analyze-first applications. These blocks—which can be powered independently when needed—enable a range of functions. (See figure 2).
Figure 2: Block diagram of the analogML core
- Sensor interfacing—interface circuitry can be synthesized for specific sensor types (microphone, accelerometer, etc.)
- Analog feature extraction—picks out salient features from raw, analog sensor data, drastically reducing the amount of data going into the neural network
- Analog neural network—efficient, small-footprint, programmable analog inferencing block
- Analog data compression—continuous collection and compression of analog sensor data supports low-power data buffering
An Analog Paradigm Shift
AnalogML goes far beyond using a little bit of analog computing for a small fraction of the overall ML chip calculations to save power. It’s a complete analog front-end solution that uses near-zero power to determine the importance of the data at the earliest point in the signal chain—while the data are still analog—to both minimize the amount of data that runs through the system and the amount of time that the digital system (ADC/MCU/DSP) is turned on. In some applications, such as glass break detection, where the event might happen once every ten years (or never), using the analogML core to keep the digital system off for 99+% of the time can extend battery life by years. This opens up new classes of long-lasting remote applications that would be impossible to achieve if all data, relevant or not, were digitized prior to processing.
The bottom line is all analog computing is not equal. No matter how much analog processing is included in a chip to reduce its power consumption, unless that chip operates in the analog domain, on analog data, it’s not doing the one thing that we know saves the most power in a system—digitally process less data.
Watch the video to learn more.
Marcie Weinstein, Ph.D. brings more than brings 20+ years of technical and strategic marketing expertise in MEMS and semiconductor products and technologies to Aspinity. Previously, she held multiple senior leadership roles in technical and strategic marketing as well as in IP strategy at Akustica, from its inception through its acquisition, after which she managed the company’s marketing strategy as a subsidiary of the Bosch Group. Earlier in her career, she was a senior member of the MEMS group technical staff at the Charles Stark Draper Laboratory in Cambridge, Mass. Connect with her on LinkedIn.