The Evolution of AI Inferencing
January 19, 2021
Story
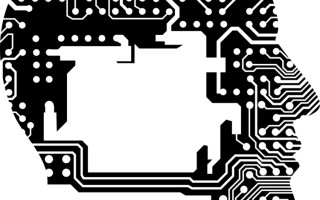
The AI inference market has changed dramatically in the last three or four years. Previously, edge AI didn’t even exist and most inferencing capabilities were taking place in data centers, on super computers or in government applications that were also generally large-scale computing projects.
The AI inference market has changed dramatically in the last three or four years. Previously, edge AI didn’t even exist and most inferencing capabilities were taking place in data centers, on super computers or in government applications that were also generally large-scale computing projects. In all of these cases, performance was critical and always the top priority. Flash forward to today and the edge AI market is starkly different than this, particularly as it enters more commercial applications. For these use cases, the main concerns are more about low cost, power consumption, and small form factors; and less about raw performance.
Balancing Better Performance with Hardware-Software Co-Design
When looking at inference chips, it’s clear that one chip is not like another. Designers are always making choices in their designs and the good ones take into consideration their end application and what constraints they have in those. As an example, when Flex Logix was designing its first inference chip, it was originally 4x the size of what it is today. We quickly realized that a chip needs to be much smaller to be player in the lower cost, lower power, smaller form factor edge AI market.
Interestingly, most people originally though that most inferencing would always be done in data centers. This view changed eventually because the industry realized it had too much data to move around and as a result, that data started moving out towards the edge. As 5G started to emerge, it was even clearer that it was impossible to send raw data to the cloud to be processed at all times. Clearly, there had to be a certain amount of intelligence at the edge that could iron out 99.9% of the scenarios and the data center really only needed to be used for extreme cases. A perfect example is a security camera. The edge AI needs to be able to figure out if there is any suspicious activity happening and if there people around. Then, if there is anything interesting found, those portions can be sent to the data center to be processed even further. However, that data sent to the data center is really just a tiny fraction of the overall inferencing. Edge inference AI is generally meant to satisfy a lot of constraints and sometimes if you want to run really large sophisticated models, you really only have run those on a very small portion of the data.
Another misconception in early edge AI inference design was that a one size fits all approach would suffice. That too proved to be wrong as specialized chips emerged that showed off their advantage and power. The key is really building chips around algorithms because you can get much better performance if it can really hone in on the algorithm. The right balance is really to get the most efficient computation just like a specialty hardware, but with programmability at compile time.
Programmability is Key
The industry is really on the cusp of AI development. The innovation we will see in this space for the next several decades will be phenomenal. And like any market with longevity, you can expect change. That is why it is becoming critical to not design chips that are super specialized for certain customer models. If we did that today, by the time the chip is in the customer’s hands two years later, the model would likely have changed substantially – and so would the customer’s requirements. That is the prime reason we keep hearing stories about companies finally getting their AI inference chips – and then finding out they don’t really perform like they need them to. That problem can be easily solved if programmability is built into the chip architecture.
Flexibility and programmability in AI is of the utmost importance today in any edge AI processor. Customers have algorithms that change on a regular basis and the system design changes as well. As Edge AI capabilities roll out in mainstream, it is going to be increasingly clear that chip designers need to be able to adapt and change to customers models instead of opting it for what they “think” the model is going to be. We see this time and time again and this is why compilers are so important. There are a lot of techniques in the compiler that are hidden from the end-user and these are around allocating resources to make sure everything is done efficiently and with the least amount of power.
Another key feature being closely looked is throughput. Good inferencing chips are now being architected so that they can move data through them very quickly, which means they have to process that data very fast, and move it in and out of memory very quickly. Often times, chip suppliers throw out a wide variety of performance figures such as TOPS or ResNet-50, but system/chip designers looking into these soon realize that these figures are generally meaningless. What really matters is what throughput an inferencing engine can deliver for a model, image size, batch size and process and PVT (process/voltage/temperature) conditions. This is the number one measurement of how well it will perform, but amazingly very few vendors provide it.
Edge AI Moving Forward
Many customers today are starved for throughput and are looking for solutions that will give them more throughput and larger image sizes for the same power/price as what they use today. When they get it, their solutions will be more accurate and reliable than competing solutions and then their market adoption and expansion will accelerate. Thus, although the applications today are in the thousands or tens of thousands of units, we expect this grow rapidly with the availability of inference that delivers more and more throughput/$ and throughput/watt.
The Edge AI market is growing quickly as is the chip suppliers vying for a position in this market. In fact, projections are that AI sales will grow rapidly to tens of billions of dollars by the mid 2020s, with most of the growth in edge AI inference. No one can anticipate the models of the future, which is why it’s more important to design with flexibility and programmability in mind.
About the Author
Geoff Tate is CEO and co-founder of Flex Logix, Inc. Earlier in his career, he was the founding CEO of Rambus where he and 2 PhD co-founders grew the company from 4 people to an IPO and a $2 billion market cap by 2005. Prior to Rambus, he worked for more than a decade at AMD where he was the senior VP of microprocessors and logic with more than 500 direct reports.
Prior to joining Flex Logix, Tate ran a solar company and served on several high tech boards. He currently is a board member of Everspin, the leading MRAM company.
Tate is originally from Edmonton, Canada and holds a Bachelor of Science in Computer Science from the University of Alberta and an MBA from Harvard University. He also completed his MSEE (coursework) at Santa Clara University.