Major Truck Fleet Manufacturer Chooses Premio Embedded Telematics Computer for Autonomous Data Routing and Monitoring
June 18, 2020
Story
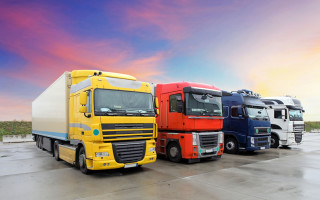
Today's artificial intelligence (AI), ADAS and sensors deliver critical data in real time that must be monitored and processed when used in autonomous fleet routing systems.
When a major U.S. truck manufacturer recognized there was a huge opportunity to mastermind the implementation of autonomous route capabilities in its fleet of trucks, it searched for embedded computing capable of handling the vast and diverse data. Today’s artificial intelligence (AI), advanced driver-assistance systems (ADAS) and sensors deliver critical data in real time that must be monitored and processed when used in autonomous fleet routing systems. This requires an industrial-grade embedded system data logger capable of providing a reliable, rugged and high-performance architectural system to support such autonomous routing.
The country’s reliance on truck fleets was clearly visible during the COVID-19 crisis. Product shortages, delivery speeds, logistics nightmares were all painfully apparent. Yet, according to the Business Insider, 71% of all freight in the U.S. is moved via trucks even during a non-pandemic year.
This case study shows how this fleet manufacturer selected and implemented Premio’s rugged computing technology to further its autonomous routing capabilities. This case study will discuss the criteria established and the successful implementation of Premio in-vehicle telematics computers in the framework of autonomous driving.
Autonomous Fleet Routing Challenges
The fleet and truck manufacturer dealt with four main challenges in its quest to support autonomous routing capabilities. It required:
- A ruggedized computing solution that withstands wide operating temperatures, shock and vibration. Although the solution is housed within a truck’s-controlled environment, the vehicles often sit out in the sun, or drive through terrain causing substantial vibration and shock — all detrimental to the electronic equipment.
- Substantial real-time decision-making capabilities. This backbone of autonomy provides telematics information on where the vehicle is and how fast the vehicle is going — data used to train autonomous driving neural networks.
- Large storage capacity for long-haul driving data and 10GbE connection to move data bi-directionally tying back to all of the telematics and improvements for future analytics. This data must be stored in a rugged computer and effectively accessed to continuously train machine learning algorithms
- A powerful multicore CPU/GPU combination that provides the capabilities for inference and real-time computations, and data telemetry
Successful implementation of autonomous fleet routing requires a mixture of diverse hardware technologies working together simultaneously. An analogy can be made as to how the human body works. For example, in the IoT space, numerous sensors are placed around and throughout the vehicle to monitor and map out the environment — the equivalent to human eyes and ears. There must be high intelligence for decision making. The data input is akin to the brain for data processing decisions in real time. Finally, vehicle telematics come into play working with the brain, acting as gatekeepers like the body’s stomach and lungs — dispersing food or oxygen into the body to make sure it continues to run.
Making sure all of these are in place and working together is no small task and requires many safety precautions. This automated trucking fleet must be reliable, able to travel on highways as well as local streets, and operate at all times, in changing weather conditions and moving environments.
The Importance of Telematics
Telematics systems provide insight into an autonomous vehicle’s speed, real-time location, fuel use, mileage, weather, road conditions and more, playing a critical role in data management. Real-time location enables delivery times to be estimated and the monitoring of road conditions, speed, health, weather, to increase vehicle efficiency and yield greater safety. They are also instrumental in predicting when the autonomous vehicle should be serviced. For fleets, telematics provides access to vehicle tracking, routing, reporting and more.
Ultimately, knowing speed, location, etc., translates into active management of fleets so fuel costs are controlled, accidents reduced, and energy efficiency capitalized upon. Items that can be managed include setting speed limits for safety and reduced fuel waste, automatic shut off based on idle time, and ensuring seat belts are fastened before the vehicle is operational.
Telematics are critical in route planning so itineraries can be sent and synchronized with each connected vendor, and GPS data can be analyzed and archived. They are also the lifeblood of the routing system the manufacturer was dedicated to employ.
What were the Criteria Established?
The trucking fleet manufacturer established a laundry list of requirements, including:
- A reliable solution addressing robust computing challenges plus demanding shock and vibration environments
- Commercial off-the-shelf industrial-grade computers that can be quickly selected and deployed for real-world usage
- Fanless computing design able to withstand wide operating temperatures and input voltage from vehicle batteries, as well as an embedded architectural structure to aggregate and monitor the valuable data and place all of that data back into a neural network.
- Able to support GPU up to RTX 2060 Super for real-time analytics and compute
- Accommodation of large storage capacities up to 32 terabytes to log the available data
What was really required, however, was not to provide the actual autonomous driving, but to support it in real time with a reliable telematics computer validated to meet the requirements for power, speed, and accuracy.
Telematics and GPU Computing — Data Collection and Analysis
Key to successful autonomous fleet routing is a telematics computer that takes the wealth of data provided by diverse sensors and indicates exactly what is happening in real time. The solution must be rugged to be able to withstand the wide range of temperatures, shock and vibration that can cause electronics reliability issues. It must also feature pinpoint accuracy as to the vehicle’s GPS location and be able to accurately push all of the data to a central database so that the telematics manager can monitor what is happening in real time.
For the autonomous fleet, Premio delivered its ACO-3011E rugged fanless in-vehicle telematics computer with an Intel Broadwell-U processor. Designed to balance performance and power, the computer features two chassis form factors accommodating versatile I/O and expansion options, making it ideal for in-vehicle applications where performance, power, and adaptability are all equally important.
Figure 1. The Premio rugged fanless telematics computer featuring an Intel Broadwell-U processor. Source: Premio
In addition to the telematics portion of the solution, Premio also delivered the VCO-6033E-2PWR Industrial GPU with an LGA 1151 socket for 6th/7th generation Intel® processor. The 6033-E provides the hardware to support inference analysis and machine learning for in-vehicle computers. For example, they perform parallel computations to process data regarding road conditions, hazards, driver maneuvers, and operation of vehicle control systems — doing so with minimal latency. They also simultaneously process data concerning weather and traffic conditions to optimize fuel consumption. This solution enables operability and responsiveness of autonomous vehicles, allowing them to continue to improve via machine learning.
Figure 2. The Premio VCO-6033E-2PWR Industrial GPU that supports inference analysis and machine learning for in-vehicle computers
For the autonomous system, Nvidia’s technology performs the actual calculations on all data that comes through the Premio system. The GPU provides the machine learning for the autonomous system, while Premio supplies the telematics computer for that data via its ruggedized hardware and GPU expansion support.
Autonomous driving really depends on edge computing and its ability to process critical data in real-time Premio’s embedded computers are certified to work with major cloud partners for IoT decentralization and its entire IoT connectivity suite . Cloud partners like Microsoft Azure IoT and Amazon’s AWS IoT Greengrass both help bridge their solutions at the edge for autonomous use cases.
Why Premio?
Premio, based on its 30 years of industrial computing expertise, worked directly with the manufacturer and R&D team, focusing on ruggedized deployment and reliability. Headquartered in Los Angeles, Premio was selected because of its embedded product offerings for in-vehicle computing and the availability of substantial local support.
Once the manufacturer’s goals were set, Premio rapidly delivered its off-the-shelf ruggedized computing units and worked closely with the manufactures’ engineering team These computers are now deployed and used successfully in driving cabins for multiple fleets trucks on their autonomous journey.
Summary
There are five levels of autonomy and the most advanced programs are testing actual miles driven, meeting Level 4 requirements. When 5G is fully implemented, however, fleet management will be in the fast lane to Level 5 autonomy. Specific 5G benefits include:
- Speed boosts of 10x, possibly over 1 Gb/s, no matter the population density
- Connection density of 100x over 4G/LTE impacting the responsiveness in accessing the network
- Real-time response with 5x to 10x lower latency rates for greater efficiency especially in vehicle safety and collision avoidance. 5G reduced latency improvement is dramatic. According to a recent CTIA white paper, it takes approximately 4.6 feet for a 4G car to apply its brakes. In comparison, a 5G car performs the task in just one inch.
Moving from Level 4 to Level 5 will require a substantial increase in miles driven and massive data aggregation for further training of AI neural networks, so there is still considerable development required.
The architecture of autonomous driving represents a mix of many hardware technologies, from IoT sensor to GPU CUDA to rugged vehicle telematics. Premio’s COTS embedded telematics data logging solutions provide the necessary infrastructure to travel the distance to Level 5.