5 Key Considerations When Choosing an Embedded Edge AI System for Smart Retail
November 23, 2021
Story
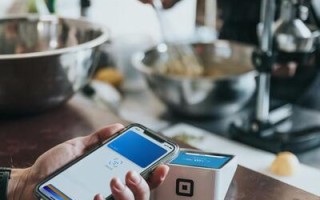
While the appearance of the COVID-19 pandemic has undoubtedly shaped consumer buying behavior, it has also accelerated pre-Covid retail trends. Trends such as contactless payments and non-traditional shopping experiences did not arise from the pandemic; however, their usage is expected to increase.
Emerging from the pandemic, brick-and-mortar retailers have no option but to respond and modernize their retail strategies or continue in the demise of pre-covid store closures. This trend presents an opportunity for software companies and other smart retail solution providers seeking to enable retailers. Promising software startups such as Trigo or Tiliter, and even industry giant Amazon, enable a frictionless retail shopping experience through computer vision, but behind these algorithms is the hardware that makes everything possible.
Follow us as we dive into the key considerations when selecting the ideal embedded Edge AI system for the smart retail environment.
-
Processor Type for AI at the Edge
Artificial Intelligence underlies all sorts of capabilities, including but not limited to, object detection, biometrics, computer vision, and a whole lot more. And while a whitepaper can be written to discuss the pros and cons of each type of processor, we will briefly cover two major processor types commonly sold by top embedded manufacturers:
CPU
The central processing unit (CPU) is commonly found in most electronic devices and is a multipurpose processor able to perform complex arithmetic, logic, controlling, and input/output operations. However, as compared to GPUs (of FPGAs), the CPU architecture is optimized for sequential serial processing through the limited number of available performance-driven cores.
When performing tasks such as computer vision, large amounts of data are required to be processed in parallel to analyze colors, patterns, shapes, etc. The volume of data can be immense, especially when considering multiple concurrent sources of high-resolution video streams. For this reason, GPUs and FPGAs can potentially be more capable than CPUs.
GPU
Graphic processing units (GPU) originated from the requirement of high-speed graphics rendering. It was later discovered that deep learning neural networks could be trained with NVIDIA GPUs, which resulted in significant performance increases. The reason is that GPUs are designed with a large number of parallel cores, delivering parallel computations for neural network training and AI inferencing.
The limitations on GPUs include a high-power consumption (although NVIDIA Jetson SoMs are designed to mitigate this issue), and while performance is generally better than CPUs, they may not deliver as much performance as the application-specific ASIC processors, but where they make up for is the support for robust SDKs and Frameworks.
-
Thermal
Probably one of the least mentioned considerations is that of thermal performance. The idea is that when the system's internal temperature rises to a certain point, the processor will throttle and reduce performance. In the case where the housing includes a fan, the resulting noise as the fan spins up may be noticeable and not ideal in certain situations.
While it is difficult to visualize the thermal performance at first glance, companies will often test and benchmark competing systems.
-
Rich IO Ports
Determining which IO port and the quantity required may seem trivial, but this is another key consideration when choosing an Edge AI embedded system. But before coming to a quick decision, we must first consider the application and the environment size. The requirements of a convenience store will vary from those of a hypermarket and those of a supermarket. The number of ports required will grow with the size of the retail space, and therefore the embedded system will need to support the necessary number of sensors plus a few more if you wish to future proof the solution.
As for the specific IO port required, from our experience in the smart retail space, most cameras will generally be IP or USB cameras.
-
Branding
The Edge AI embedded system represents the brand of your company and can offer a memorable impression. Your brand allows you to differentiate from competitors and shape the narrative of what your brand represents. Whether the device is in plain sight or hidden behind a panel, it would be ideal to match your brand's color and design with that of the system. After all, would you really want to show a manufacturer's standard blue or beige colors over your own brand colors?
-
Unwavering Support
Challenges are bound to arise along your AI project life cycle, especially when working with an unknown SDK or BSP. To quickly overcome these hurdles, it's critical to have a knowledgeable partner that can offer timely support. Nothing is more bothersome than having to wait a week for a response from your partner and potentially delaying the project.
Choosing a partner who has proven experience handling these technical issues can be the difference between timely and delayed deployment. In addition, the relationship between chipmakers and their partners varies depending on the partnership tier, thereby affecting the speed at which you can expect a response from your hardware partner. For example, an NVIDIA Elite partner may solve your issue within a couple of days, whereas an NVIDIA Preferred partner may take a week.
So how does this work in practice?
So now that we have a basic understanding of the considerations for selecting an Edge AI embedded system, let's see how this works in practice.
For a situation where a target customer's retail space was a convenience store wishing to enable a frictionless shopping experience with three existing IP cameras, you may consider an Edge AI system such as AVerMedia's NX215B, which includes an NVIDIA® Jetson Xavier™ NX module. This high-inference multicore processor supports multiple sensors while being power efficient.
The embedded system includes a number of Ethernet ports (in addition to HDMI, USB3.0, and a 20pin connector), an SSD, and WiFi, while maintaining a small footprint, ideal for the smaller retail space. And as an NVIDIA Elite partner, their support is guaranteed to be timely for any inconveniences that may arise.
Of course, if you require that bright neon green branded color, I'm sure they can customize that for you too.
Jeremy Juan, Senior Marketing Manager of AVerMedia, has extensive experience in multiple aspects of the IPC industry, including embedded edge AI and rugged computing, from his many years in the industry's big-name companies. He has personally directed and executed the launch of a number of award-winning flagship products towards key global markets