STMicroelectronics Streamlines Machine-Learning Software Development for Connected Devices and Industrial Equipment with Upgrades to NanoEdge AI Studio
December 03, 2021
News
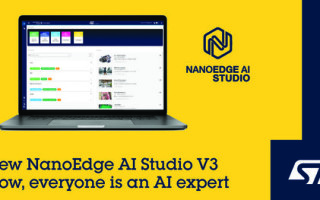
STMicroelectronics announced the availability of Version 3 of NanoEdge AI Studio, the first major upgrade of the software tool for machine-learning applications that ST acquired with Cartesiam earlier this year.
The new version of NanoEdge AI Studio comes as the shift of AI capabilities from the cloud to the edge offers manufacturers the potential to fundamentally improve industrial processes, optimize maintenance costs, and deliver functions in equipment that can sense, process data, and act locally to improve latency and information security. Applications include connected devices, household appliances, and industrial automation.
NanoEdge AI Studio is designed to simplify the creation of machine learning, anomaly learning, detection, and classification on any STM32 microcontroller. This new release also includes prediction capabilities such as regression and outliers libraries. The tool is designed to make it easier for users to integrate machine-learning capabilities quickly, easily, and cost-effectively into their equipment. Per the company, no data-science expertise is needed.
Adding native support for all STM32 development boards, ST has also eliminated the need to write code for its industrial-grade sensors with new high-speed data acquisition and management capabilities. NanoEdge AI Studio software enhances security by using local data storage and processing, instead of transferring to, and processing data in, the cloud.
Key Features of NanoEdge AI Studio V3:
- Completely redesigned user interface for non-experts to develop machine-learning libraries.
- New high-speed data acquisition and management on the STWIN development board making all industrial-grade sensors manageable without having to write a single line of code.
- Improved support for anomaly detection, particularly useful for predictive maintenance to anticipate wear-and-tear phenomena or to better deal with equipment obsolescence.
- Learn normality directly on STM32 MCUs using small dataset or use new algorithms to train on without ever seeing abnormal patterns before.
- Added regression algorithms to extrapolate data and predict future data patterns for energy management or forecasting remaining life of equipment.
- Native support of all STM32 development boards, no configuration required.
For more information, visit the ST website.