Expert Predictions for 2020 Part 4: AI and Machine Learning
December 27, 2019
Blog
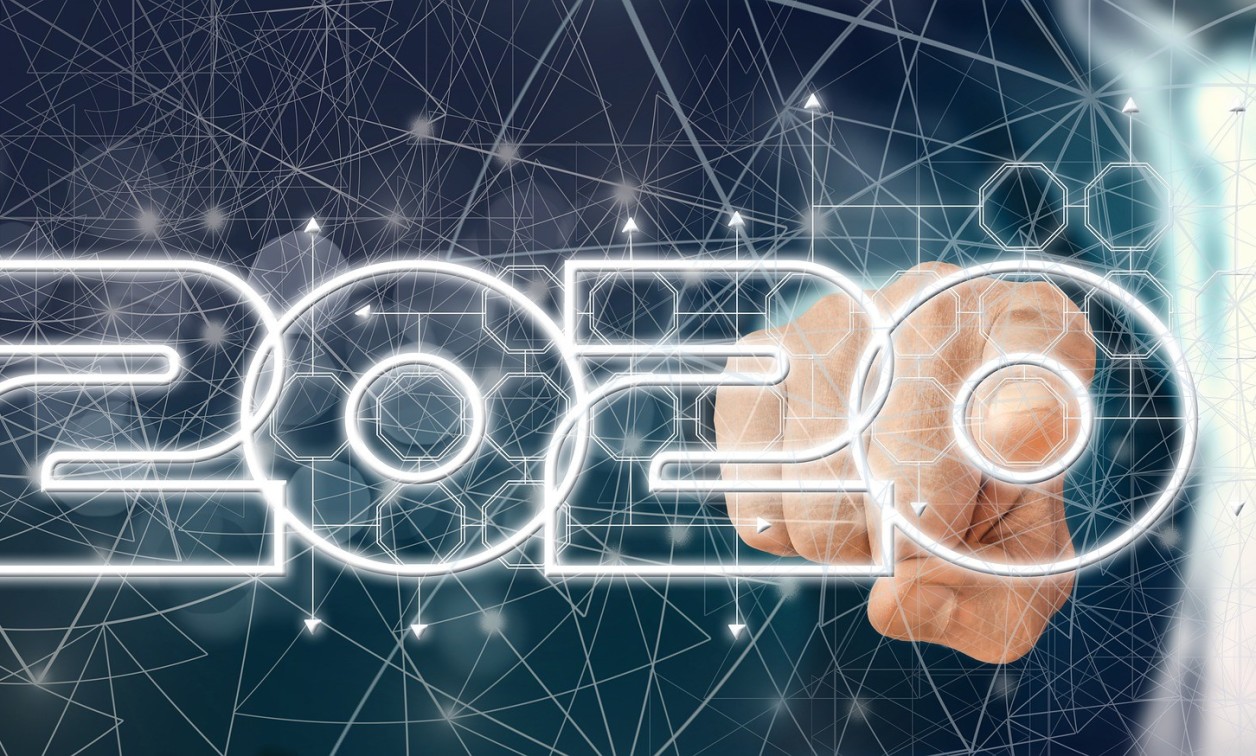
In part four of our five-part series, experts from Western Digital and MathWorks share in detail their thoughts on the future of AI and Machine Learning.
With 2019 quickly coming to an end, the industry is looking ahead to 2020. At this point, only predictions can be made about the hot topics that surround the technology world. In this blog series, industry experts share their thoughts and predictions heading into the new year. We’ll be covering the key technology categories of edge, cloud, 5G, artificial intelligence, and the evolution of engineering.
In part four of our five-part series, experts from Western Digital and MathWorks share in detail their thoughts on the future of AI and Machine Learning.
Steve Phillpott, CIO, Western Digital
Companies will Begin Automating Data Scientist Roles for ML
There are simply not enough data scientists in the world to support the growth of ML workloads. Companies are now developing ways to put the power of ML into the hands of software engineers and/or business subject matter experts. New off-the-shelf tools will be able to fulfill the baseline role of the data scientist, and true data scientist roles will shift to higher-level value-add such as fine-tuning ML for specialty use-case work. In 3-5 years, ML automation will become the norm, and companies will have more tools at their disposal to empower data scientist personnel to be more efficient and agile.
AI/ML Will Help Drive the Adoption of “Auto” Everything
In IT, the quest for greater efficiency is never-ending, and automation is essential in succeeding. From database tasks to manufacturing to cars to customer experiences and self-service business functions, virtually anything and everything that can be automated, will be. New AI/ML models and insights, leveraging the convergence of multiple data types, will be the key enablers of automation. In 2020, organizations will continue to implement AI/ML in order to unlock the power of automation to drive efficiency and increase productivity. The result? Reduced costs across the value-chain.
We’ll See more Standardization in the AI/ML Ecosystem that Makes it Easier to Integrate and Deploy Solutions at the Edge
Analytics are the competitive advantage – companies that aren’t investing in analytics by 2020 probably won’t be in business in 2021. There is just too much company data that can be collected, processed, and then turned into insights. Those that do not take full advantage of modern analytic tools in this space will suffer. With this, the new connected world has more and more of the workloads moving to the edge, increasing the need to ensure these very small edge devices have the capability to run and analyze increasing large amounts of data. Due to the small footprint and the rapid deployment needs, we’ll need to have more standardization and interoperability. This will take the form of more open architecture, open standards, open messaging and more.
Philipp H. F. Wallner, Industry Manager, MathWorks
AI Becomes an Enabler of Flexible Production
For the past several years, the automation industry has discussed a vision of “sample size one” – how can production lines produce one-of-a-kind goods without encountering long changeover-times or other inefficiencies. With Industry 4.0, this vision must come true to meet the requirement of full individualization in production. This means machines cannot be set up in a fixed, inflexible manner on the shop floor, i.e. commissioned, parameterized and tuned for one specific product produced repeatedly for months or even years. Production lines must be flexible. They must be built from multiple mechatronic modules that can easily be rearranged. There must also be an AI that parameterizes and tunes machines according to the next, individualized good manufactured on the line.
Edge Computing will Help AI and Predictive Maintenance Evolve
The rapidly increasing calculation power of industrial controllers and edge computing devices, as well as the use of cloud systems, help achieve a new dimension of software functionality on production systems. AI-based algorithms will better optimize the throughput of the entire production line while minimizing the consumption of energy and other resources. Predictive maintenance will evolve and consider data not only from one machine or site but across multiple factories and across equipment from different equipment vendors. Depending on the requirements, these AI-based algorithms will be deployed on non-real-time platforms as well as on real-time systems like PLCs.