Applications and Benefits of Edge AI
March 08, 2022
Blog
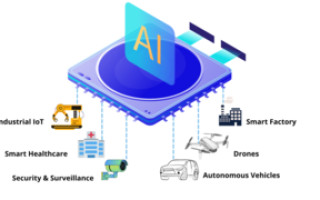
Edge AI is the combination of edge computing and edge intelligence to run machine learning tasks directly on end devices. It generally consists of an in-built microprocessor and sensors, while the data processing task is completed locally and stored at the edge node end. The implementation of machine learning models in edge AI will decrease the latency rate and improve the network bandwidth.
Edge AI helps applications that rely on real-time data processing by assisting with data, learning models, and inference. The edge AI hardware market valued at USD 6.88 billion is expected to reach USD 39 billion by 2030 at a CAGR of 18.8%, as per a report by Valuates Reports.
The advancement of IoT & adoption of smart technologies by consumer electronics and automotive, among others, are fuelling the AI hardware market forward. Edge AI processors with on-device analytics are going to enhance the opportunities for the AI hardware market. NVIDIA, Google, AMD, Lattice, Xilinx, and Intel are some of the edge computing platforms providers for such cognitive AI applications design.
The advancement of emerging technologies such as deep learning, AI hardware accelerators, neural networks, computer vision, optical character recognition, natural language processing, etc., opens new horizons of opportunities. While businesses are rapidly moving toward a decentralized computer architecture, they are also discovering new ways to use this technology to increase productivity.
What is Edge Computing?
Edge computing brings the computing and storage of data closer to the devices that collect it, rather than relying on a primary site that might be far away. This ensures that data does not suffer from latency and redundancy issues that limit an application's efficiency. The amalgamation of machine learning into edge computing gives rise to new, resilient, and scalable AI systems in a wide range of industries.
Some wonder whether edge computing will suppress cloud computing — this is not the case. Instead, the edge will complement the cloud environment for better performance and leverage machine learning tasks to a greater extent.
Need for Edge AI Hardware Accelerators
Running complex machine learning tasks on edge devices requires specialized AI hardware accelerators that boost speed and performance and offer greater scalability, maximum security, reliability, and efficient data management.
VPU (Vision Processing Unit)
A vision processing unit is a sort of microprocessor aimed at accelerating machine learning and AI algorithms. It balances edge AI workload with high efficiency and supports tasks like image processing, similar to a video processing unit used with neural networks. It works on low power and high-performance precision.
GPU (Graphical Processing Unit)
A GPU is an electronic circuit capable of producing graphics for display on an electronic device. It can process multiple data simultaneously, making them ideal for machine learning, video editing, and gaming applications. With their ability to perform complex machine learning tasks, they are being extensively used in mobiles, tablets, workstations, and gaming consoles.
TPU (Tensor Processing Unit)
Google introduced the Tensor Processing Unit (TPU), an ASIC for executing machine learning algorithms based on neural networks. It uses less energy and operates more efficiently. Google Cloud Platform with TPUs is a good choice for ML applications that don't require a lot of cloud infrastructure.
Applications of Edge AI Across Industries
Smart Factories
Edge AI can be applied to predictive maintenance in the equipment industry, by which edge devices can perform analysis on stored data to identify scenarios wherein a failure might occur before the actual failure happens.
Autonomous Vehicles
Self-driving vehicles are one of the best examples of incorporating edge AI technology into the automobile industry, where the integration helps detection and identification of objects and significantly reduces the chance of accidents. It aids in avoiding collision with pedestrians or other vehicles and detecting roadblocks, which requires real-time data processing.
Industrial IoT
With computer vision enabled for Industrial IoT, visual inspections can be done without much human intervention, thereby increasing operational efficiency and improving productivity in assembly lines.
Smart Healthcare
Edge AI in wearables can enhance surveillance of a patient's health and forecasting early disorders. These details can also be used to provide patients with effective treatments in real-time. Patient data can be secured with HIPAA compliance in place.
Benefits of Using Machine Learning at Edge
- Higher Scalability — As interconnected IoT device demand increases, edge AI is becoming an absolute choice due to its efficient data processing without relying heavily on a cloud-based centralized network.
- Data Protection & Security — Since edge devices are not completely dependent on cloud resources, attackers cannot bring the whole cloud data center/server system to a standstill point.
- Low Operational Risks — Edge AI is based on a distributed model, so potential failure will not affect the entire system chain as in with centralized cloud models.
- Reduced Latency Rate — Edge AI computation can be performed in milliseconds by eliminating the need to send data to the cloud for initial processing.
- Cost-Effectiveness — Data transfer is minimized with edge AI, which saves a lot of bandwidth. This also reduces the capacity requirements for cloud services, making edge AI a cost-effective solution when compared to cloud-based ML solutions.
In several instances, machine learning models are large and complex, which can make it extremely difficult to shift them to compact edge devices. Without proper precautions, reducing the complexity of the algorithms can take a toll on the processing perfection and computation power. It's crucial to evaluate all the failure points in the initial development stage. Priority should be given to testing the trained model perfectly on different types of devices and operating systems.