Building, Training AI models Needn't Be Confusing and Time Consuming
August 11, 2020
Story
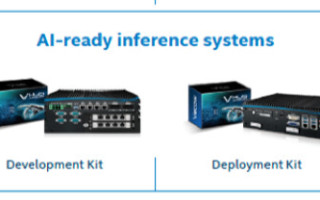
As the technology gets pushed out to the Edge of the IoT, the number of uses climbs considerably. Developers are moving quickly toward deployment of their AI architectures.
It wouldn’t be much of a stretch to say that artificial intelligence (AI) can be used in just about any application in the industrial sector. As the technology gets pushed out to the Edge of the IoT, the number of uses climbs considerably. Developers are moving quickly toward deployment of their AI architectures, thanks to advances from vendors like Vecow.
The days of having to program AI devices manually are thankfully in the past. As a result, the speed of deployment is increasing while the cost is shrinking. While it’s getting easier, designing an optimal model for a specific AI scenario can still be time-consuming and challenging.
The most difficult part of the design process is training the AI model to provide core capabilities like object detection, motion tracking, and facial recognition. That training can impact system cost: the more efficient the model deployed, the fewer the resources that are required to implement it.
Vecow’s VHub AI Developer features an integrated solution that reduces model training time and provides the resources required for engineers to develop their Edge-based AI solutions. Four versions are available, ranging from a starter kit with an Intel NUC (Next Unit of Computing), which is based on an Intel Core processor, up to the Titan Kit, which offers a choice of an Intel Core SoC or an Intel Xeon processor for compute-intensive applications. All versions include a labeling tool, a training platform, an inference solution, and more than 200 pre-trained models for typical Edge use cases.
A Complete Framework for Edge-Based AI
The VHub AI Developer provides a complete development framework for Edge-based computing applications. The kit is relatively easy for a seasoned developer to deploy, and it’s compatible with most platforms and includes a set of more than 200 scalable AI models. The applications covered by those models include common functions like object tracking, facial recognition, and motion detection.
As a result, system integrators can focus on developing and training the AI model, rather than spending their time integrating and maintaining the entire AI framework. Pre-integrated and pretested software tools further streamline the process.
(The VHub AI Developer is available in four different versions, as shown in the figure.)
The four different versions of the VHub AI Developer help provide the best combination of hardware and software resources for a particular application (see the figure). The VHD NUC Series is a basic starter kit; the VHD ECX-1000 PoER Series Deployment Kit brings rich I/O capabilities; the VHD ECX-1400 PEG Series Deployment Kit introduces a GPU computing engine; and the VHD RCX-1520R PEG Series Titan Kit delivers even more GPU capabilities for the most compute-intensive applications.
In all versions, the framework has been integrated and tested, further reducing development time. In addition, the VHub AI Developer framework is designed to guarantee stable version management, so the design should never suffer from version control issues, a common occurrence in open-source AI training tools.
Use Cases
Machine vision and automation are two popular use cases for AI, and hence, for the VHub AI Developer. Smart retail and access control are also prominently employed. Here’s why the tool stands out for each:
- Machine vision: Efficiency and accuracy are critical for classifying defective parts in factories. Preinstalled inspection SDKs with VPU and GPU accelerators enable high accuracy at a low cost.
- Automation: Intelligent automation integrates smart technologies and services to carry out critical tasks. With a preinstalled automation monitoring SDK, manufacturers can enhance productivity.
- Smart retail: Retail stores need to know and understand their customers to increase revenue and profitability. A pre-installed feature recognition SDK lets engineers capture gender, age range, customer count, and in-store behavior to create targeted experiences.
- Access control: Security often depends on granting access only to authorized users. Using facial recognition, data can be stored in a vision library to quickly and conveniently approve or deny access.