Innovation in sensor analytics at the edge
April 28, 2017
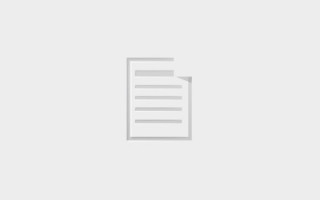
The Industrial Internet of Things (IIoT) revolution is in full development as industrial providers continue integrating smart connected solutions into...
The Industrial Internet of Things (IIoT) revolution is in full development as industrial providers continue integrating smart connected solutions into their existing operations. A high level challenge is that many IIoT solutions are tasked with capturing and moving massive amounts of data from a myriad of sensors to the cloud. Often, this creates an inadvertent crippling effect from a bottleneck deluge of raw data. With a dumb sensor approach, the typical “send everything to the cloud” model can be drastically inefficient. This often presents cloud platforms with the near impossible task of extracting a few morsels of valuable data from an overwhelming amount of noise.
Within the IIoT, the role of precision sensing and measurement are critical in applications where reliability and accuracy are paramount. IIoT innovators face several challenges in managing high volumes of data while also maintaining integrity throughout the solution. System engineers are leveraging both hardware and software innovation at the edge to overcome these challenges, with one solution being smarter sensor nodes capable of distilling and interpreting data at the edge so that only important data is sent to the cloud for processing. A model of distributed analytics processing enables localized decision making and alleviates many of the concerns surrounding latency, security, bandwidth, and power consumption.
Optimized smart partitioning maximizes data value
Since only a subset of IIoT data is truly valuable, a large majority of the data is unimportant and can be ignored. However, the highest quality sensed data can still be marginalized without careful attention to an edge node’s analytics requirements.
System data that requires real-time local decisions should be acted upon long before it is aggregated at a remote point in the cloud. In contrast, information that leverages historical value with prediction models to influence long-term insights makes an ideal application for cloud processing. Archiving data into mass databases for retroactive processing and decisions plays to the strengths of powerful cloud processing and storage.
Edge processing can be an analytic proposition that allows data to be analyzed close to its source in addition to being sent it to a remote server for cloud-level analysis. Through close coupling to the sensor, time-sensitive feedback loops can provide immediate processing that provides more informed decisions, while moving real-time analytics processing as early as possible in the signal chain also reduces the payload burden downstream and shortens latency. Furthermore, initial data processing at the edge node can simplify data formatting and reduce communications bandwidth for eventual aggregation at the cloud gateway (Figure 1).
[Figure 1 | Smart partitioning at the edge node enables leaner processing and intelligence further ahead in the signal chain allows for a more efficient IoT solutions.]
Processing for edge node insights – The smart factory
One application that will be important within the IIoT is machine vibration condition monitoring. New or legacy machine equipment can have key mechanical components (such as rotating shafts or gears) mounted with high dynamic range MEMS accelerometers. These multi-axis sensors sample the vibration of machinery in real-time, and these signatures can be measured, processed, and compared to an ideal machine profile. In a factory, the analysis of this information aids efficiency, reduces line-down situations, and can predict mechanical failures in advance. In extreme cases, a machine with a rapidly deteriorating mechanical component that would otherwise induce further damage can be immediately shut down.
The intent of the solution is to identify and predict machine performance issues in advance of failure. At the edge sensor node, a multi-axis high dynamic range accelerometer monitors vibration displacement at various locations on industrial machines. The raw data can be filtered and decimated for frequency domain interpretation within a microcontroller (MCU). A fast Fourier transform (FFT) compared against known performance limits can be processed for testing against pass, fail, and warning alerts downstream (Figure 2). Processing gain within the FFT can be achieved through finite impulse response (FIR) filtering to remove wideband noise that is otherwise outside the bandwidth of interest.
[Figure 2 | Though machine maintenance can be performed routinely, it is often not done with intelligence about a machine’s condition. By analyzing the vibration performance of specific machine operations, edge node alerts can provide predictive points of failure and maintenance milestones.]
Edge node processing is an important component in machine condition monitoring. The full bandwidth of sampled data can provide a significant aggregation bottleneck at a wireless gateway, considering that a single machine may have many sensors and hundreds of machines may be monitored concurrently. Filtering and intelligent decision making within an MCU offers a low-bandwidth output to the wireless transceiver without the need for intensive filter processing in the cloud (Figure 3).
[Figure 3 | Typical signal chain for vibration monitoring.]
Decision time latency can be drastically reduced by edge node analytics. An example of this can be seen in Figure 4, where a MEMS sensor warning threshold limit is exceeded and an alert is immediately sent. If the event is extreme enough to be deemed critical, the node may be given authority to automatically disable the offending equipment to prevent a time-sensitive catastrophic mechanical breakdown.
[Figure 4 | A time domain representation of sampled machine vibration data where a comparator threshold can determine whether or not the sensed and measured data is communicated beyond the edge. A lower power state can be maintained to filter a majority of the information until data preponderance is achieved by a threshold-crossing event.]
Alternately, a trigger signal may be invoked to enable another sense and measurement node (such as one on a secondary machine component) to begin interpreting data based on the first event, reducing the total data set of sampled data from the edge node. In order to determine any vibration abnormality, the front-end node must be designed with the required detection performance. The dynamic range, sample rate, and input bandwidth of the sense and measure circuit should be more than sufficient to identify any excursion event.
Interpretation
If mechanical signature frequencies of interest are known precisely, the sample rate of the analog-to-digital converter (ADC) and FFT size within the MCU can be planned such that the maximum amount of energy falls within the width of a single histogram bin. This will prevent signal power from leaking across multiple bins and diluting the precision of the amplitude measurement.
Figure 5 is an example of an FFT where specific predetermined zones are interpreted within the edge node MCU for more than one observed mechanical component. Bin energy that peaks within the required green zone represents satisfactory operation, while the yellow and red zones indicate warning and critical alarms, respectively. Instead of transmitting the full sensor bandwidth, a lower data rate alarm or trigger breadcrumb can alert the system of an excursion event within the zones of interest.
[Figure 5 | FFT bin energy can be used to trigger alarms.]
Machine condition monitoring is just one example of a smart factory application where edge node processing is advantageous for IIoT system success. The most vital data can be processed and interpreted for immediate localized action. This alleviates the full bandwidth requirements burden of remote cloud analytics.
Analog Devices Inc.
LinkedIn: www.linkedin.com/company/3450
Facebook: www.facebook.com/AnalogDevicesInc
Google+: plus.google.com/+analogdevicesinc