A Non-Intrusive System to Monitor Driver Vigilance
February 16, 2021
Story
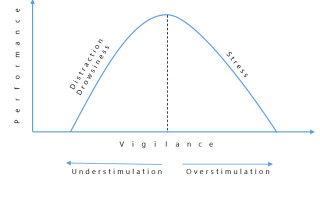
Do you think driving is a dangerous activity? It definitely is.
According to WHO (World health organization), motor vehicle crashes kill 3000 people per day. In 2018 alone, 2841 people were killed in motor vehicle crashes involving distracted drivers.
Among the whole driving population, certain categories of drivers is at high risk:
-
In 2017 young drivers, male and female, were speeding at the time of the fatal crashes more than other age groups.
-
Drivers age 70 and older have higher fatal crash rates per mile travelled than middle-aged drivers.
Medical conditions such as sleep apnoea can also increase the risk. Driver state such as drowsiness, distraction, and mood can also increase risk by affecting a driver’s information processing ability and change his or her risk-taking tendencies. Smart personal devices and infotainment systems also contribute to the road crash since the latter adds to the already demanding driving activity.
In response, automobile manufacturers are continuously searching for innovative solutions to support the driver. In the past decade, there has been exponential growth in the field of signal processing and human factor communities to develop a driver monitoring system that can sense the driver state and offer appropriate timely feedback.
Yerkes–Dodson law
A driver’s state directly affects his or her driving capabilities. This was best defined by the Yerkes-Dodson law. This law, when adapted to the driver’s state, projects an inverted U function between the driver’s arousal and his or her driving performance.
As shown in Figure 1, both high and low arousal results in poor driving performance. The optimal performance occurs when there is a good amount of vigilance to keep the driver alert but not stressed.
(Figure 1. Driver Performance as a function of Vigilance)
For example, a driver who is drowsy or has lost vigilance due to unchanging conditions, such as driving on a straight road with less or no traffic, is susceptible to losing control of the vehicle or failing to respond at the right time. Whereas strenuous driving conditions, such as heavy traffic or poor visibility, or additional workload from a secondary task (e.g., work on the navigation system or a heated phone conversation) can stress the driver and reduce the cognitive abilities.
A driver’s facial and body expressions can be used to detect a driver’s vigilance level. For example, percentage of eye closure (PERCLOS), eye blink, and yawning are useful for detecting fatigue and drowsiness, whereas eye movements, gaze direction, and head movements help in telling if the driver is attentive. These methods are heavily reliant on video processing; hence, it wasn’t possible earlier due to computational limitations. But recent advancements in computational power and algorithms have made it possible in real- time.
Inside Driver Monitoring Systems
A typical driver monitoring system includes built-in infrared (NIR), light-emitting diode (LED) diode sensors, and a charged coupled device camera positioned towards the driver. The system tracks eye movement and detects eyelid and head motion to predict drowsiness and send alerts.
(Figure 2 Driver Monitoring System)
A camera with an active lighting source is used to remove high illumination during driving. Facial and body landmarks are then detected by using tracking algorithms. Thereafter, the characteristics such as PERCLOS, head pose, and gaze direction are extracted. In the end, the system looks at patterns and derives a decision.
Generally, there are two methods of tracking the driver’s upper body movement:
-
The rigid three-dimensional head model and front face orientation detection using the facial landmark.
-
They can also be detected based on data of visual indicators such as frequency or standard deviation in a certain time interval.
The main exercise for this system is to identify the required features in every frame in real-time. These features can be the face, eye, or other facial landmarks. The software detection algorithm mostly relies on the image acquisition hardware used.
The Dark and Bright Pupil Effect
Visual indicators for drowsiness detection such as the eye area (pupil, eyelid, etc.) can also be calculated. These are used for gaze estimations and head pose estimation.
Camera eye-tracking systems with near-infrared sources are used to exploit the optical property of the human pupil. It’s called as dark and bright pupil effect. When the illumination is on the same axis with gaze, pupils reflect back to the source and appear bright in the captured image, while an offset of illumination placement will not generate such an effect and will cause dark pupils. For driver monitoring system a computationally cheap method of subtracting the alternating dark pupil frame from the bright pupil frame is performed. Though this technique is highly accurate in labs, its heavy reliance on the hardware setup reduces its robustness during real driving conditions.
(Figure 3 Eye Gaze Estimation)
With advancements in machine learning algorithms, it’s very much possible to develop intelligent computer vision solutions with large video data input for real-time applications that do not rely on image acquisition hardware configurations. Tracking algorithms are used for enhancing the processing speed of the system too.
New-gen cameras are equipped with abilities beyond human vision and can capture the smallest of changes. These cameras can enable potentially non-intrusive evaluation of driver physiology. Facial features and body movements such as head and hand positions are being used to determine the driver’s state. For example, using a cellphone, smoking and eating, etc. can be detected by tracking the hand. It is important to note that the driver’s facial and body expressions also give the means to determine the driver’s emotions.
Conclusion
The evolution of sensing technologies and the progress in our understanding of human characteristics affecting driving performance gives a scope of development of better driver monitoring solutions. These systems use facial and body expressions and psychological signals to monitor driver conditions, and also sense the environment in real-time, to provide feedback to the driver. The vehicle can also take control if needed.
During the transfer of control from the driver to the vehicle, DMS can play an important role in the timely and accurate engagement of the automatic safety systems. In these cases, understanding the driver’s intentions are of utmost importance. Once the vehicle gains control due to driver drowsiness, the DMS can actively monitor the driver by measuring his/her facial and body expressions.
A major challenge in the development of advanced driver monitoring system is the interdisciplinary nature of this field, which requires a close partnership between the researchers and practitioners in both signal processing and human behavior.
References
-
E. Murphy-Chutorian and M. M. Trivedi, “Head pose estimation and augmented reality tracking: An integrated system and evaluation for monitoring driver awareness,” IEEE Trans. Intell. Transport Syst., vol. 11, no. 2, pp. 300–311, 2010.
-
Q. Ji, Z. Zhu, and P. Lan, “Real-time nonintrusive monitoring and prediction of driver fatigue,” IEEE Trans. Veh. Technol., vol. 53, no. 4, pp. 1052–1068, 2004.
-
L. M. Bergasa, J. Nuevo, M. A. Sotelo, R. Barea, and M. E. Lopez, “Real-time system for monitoring driver vigilance,” IEEE Trans. Intell. Transp. Syst., vol. 7, no. 1, pp. 63–77, 2006.