Industry 5.0 Has Arrived, Thanks to the AMR
April 28, 2021
Blog
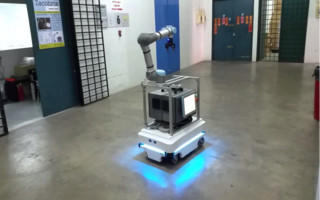
Note: This is the third in a series of four blogs (and associated podcasts). In the first blog, I looked at what it means to be operating a platform at the edge of the AIoT, where designers should start the process, and what path they should take to develop those systems. In the second, I went through some of the subsystems that go into that AIoT platform, drilling down into the nuts and bolts.
We spend a lot of time discussing artificial intelligence (AI) at the Edge of the IoT, which you now probably know is referred to as AIoT. One key element of a successful AIoT offering is an autonomous mobile robot (AMR). When operated in a factory setting, the benefits can be huge. AMRs can work 24/7/365, having to stop only to recharge their batteries or for maintenance.
To some, the use of AMRs in the factory, where they would potentially interact with humans, is the definition of Industry 5.0. While it might seem obvious that robots and people need to be in the same environment, keeping both safe and secure requires some use of AI in the AMR.
While the benefits of AMRs are obvious, the complexity that’s required to design and build them is also quite large. It starts with a vision system, and we know that whenever imaging is involved, the amount of data that comes into play can be huge.
Other aspects to consider include a precise navigation system, motor control, and wireless communications. There also should be some form of human machine interface (HMI), assuming there is interaction between the AMR and the human.
Powering Your AMR
Another important, and sometimes overlooked technology is the ability to power the AMR. In just about every case, the robot needs to be untethered and must operate for a reasonable time period before requiring a recharge.
Other critical design considerations include how you choose a capable platform to handle all these tasks, based on what kind of control that’s required. One potential starting point is the NVIDIA Jetson edge AI platform, which the company claims is “the AI platform for autonomous everything.” The Jetson is a complete system-on-module (SOM), with CPU, GPU, PMIC, DRAM, and flash storage. That could/should be combined with NVIDIA's Isaac robotics platform, including the Isaac SIM, that’s used for simulation and training of robots in virtual environments before deploying the AMRs in the real world. It also includes the Isaac SDK, an open software framework for efficient processing on the NVIDIA Jetson platform, which makes it easier to add AI for perception and navigation. It also allows for the addition of custom behaviors and capabilities, accelerating robot developments that normally take months, if not years, of engineering effort.
“NVIDIA provides a platform that serves as a great starting point,” says Zane Tsai, Director of the Platform Product Center at ADLINK Technology. “It provides the needed control along with computing efficiency for all aspects of the robot. But it’s the power efficiency that really makes it stand out.”
Lots of Sensors
The AMRs need to incorporate various sensors, ranging from 2D cameras, time-of-flight sensors, LIDAR devices, and inertial measurement units (IMUs), connecting them with high-speed I/O. And all the data from the sensors must be processed in real time on the robot itself to allow it to autonomously navigate in the complex and dynamic factory environment. And in theory, because of the integrated AI functionality, upgrades should be happening dynamically all the time. So field upgrades would be minimized.
According to Amit Goel, the Director of Product Management for autonomous machines at NVIDIA, “The simulation environment is a key component in the development and deployment of AMRs. Developing the actual hardware adds some constraints and affects how quickly they can be designed, and frankly, how many people can be working on the AMR at the same time. Once you move the design into simulation, your development team can be anywhere.”
One question that designers now face is what type of processor should be deployed, or should it be a combination of available processors. While there’s no simple answer, the assumption is that if the AMR needs to accept, read, and calculate information about its environment, then control its behavior, a GPU makes the most sense. The processor should also offer the flexibility to upscale (and potentially downscale).
To hear more detail about designing AMRs, specifically using ADLINK Technology platforms and NVIDIA GPUs, I suggest you check out the podcast below, featuring the two experts quoted here.