Revolutionizing Vision AI Applications with FPGA Technology
November 06, 2024
Sponsored Blog
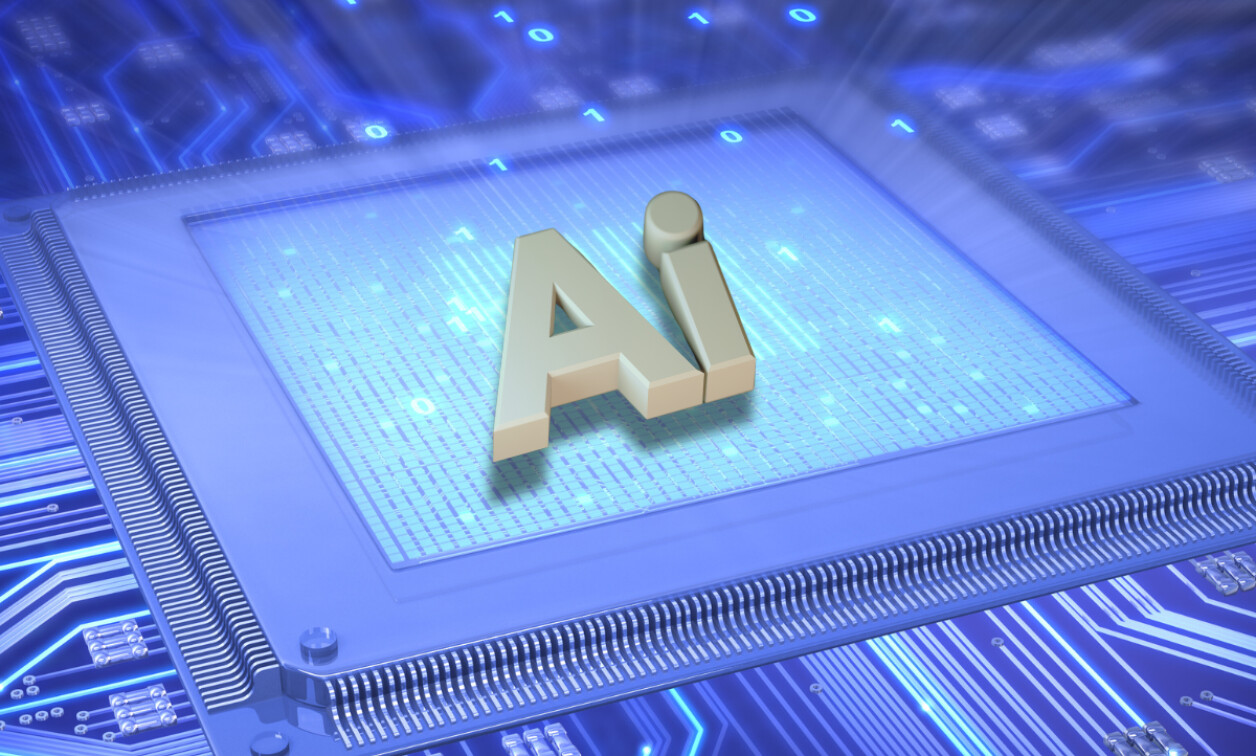
As a key player in the FPGA landscape, Enclustra is actively driving innovation in Vision AI applications. Embedded vision AI applications are revolutionizing industries by enabling highly complex real-time video stream processing and decision-making at the edge. These applications range from autonomous driving to smart manufacturing, where rapid analysis of visual data is critical. They demand real-time processing, low latency, and low power consumption to effectively analyze and interpret visual data.
The Challenge
Selecting the right hardware platform is crucial in meeting the tight requirements of embedded AI applications. The requirements involve achieving high-performance video processing with minimal and deterministic latency while maintaining low power consumption. Another requirement of the AI at-the-edge applications is the interconnectivity with multiple sensors and other peripheral devices. Traditional processors, such as CPUs and GPUs, often struggle to meet these requirements; CPUs, designed for sequential processing, can not provide the processing bandwidth of a parallelized processor architecture; GPUs, even though based on highly parallelized architecture, struggle to achieve power efficiency and ultra-low, deterministic latency; neither technology offers sophisticated sensor-fusion capabilities. ASICs, although able to achieve ultra-low latency, high power efficiency and sensor fusion capability, are not suited for applications that require flexibility to adapt to constantly evolving AI algorithms.
The Solution
FPGA technology offers a convincing solution to the challenges of the embedded vision AI:
- Parallel Processing: FPGAs can handle multiple data streams simultaneously, which makes them the perfect hardware platform to implement highly parallelized AI models.
- (Ultra) Low Latency: By having the full control of algorithm implementation at the hardware level, FPGAs enable implementing designs with ultra low latency, a critical factor in applications where split-second decisions are essential.
- Power Efficiency: Customizable logic blocks in FPGAs and little processing overhead allow for optimized power consumption, making them ideal for AI at-the-edge and power-critical applications.
- Flexibility: FPGAs can be reprogrammed over-the-air to accommodate the evolving AI algorithms without the need for hardware redesign, ensuring long product life cycles.
- Sensor Fusion: With a large number of I/Os of various types, supporting various data protocols, FPGA devices are able to connect directly to a multitude of sensors and other peripheries, simplifying the system architecture and making data protocol adapters, level shifters, etc. obsolete.
Example Application
To demonstrate the capabilities of embedded AI on FPGA technology, the Enclustra Design Services team implemented a vision AI application on an Enclustra FPGA/SoC module, Mercury+ XU7, and Enclustra Mercury+ ST1 base board. Enclustra team combined two available AI models, image classification (YoloV3-based) and car colour detection in a sequence, to achieve maximal algorithm performance. The models were implemented using the AMD Vitis™ AI toolchain, which translates AI models for optimal implementation in FPGA/SoC fabric and enables optimal usage of SoC resources.
In summary, FPGA technology’s unique mix of performance, efficiency, and adaptability positions them as the preferred hardware choice for next-generation embedded AI applications. Enclustra team’s deep experience in FPGA technology and FPGA development tools transforms the initial idea into reality.
Vision AI application on an Enclustra FPGA/SoC module and base board combination (Mercury+ XU7 and Mercury+ ST1)
Two AI models in a sequence: YoloV3-based image classification and car color detection
To learn more about Enclustra products, visit here.