Edge Analytics Complementing Cloud Computing
June 18, 2020
Story
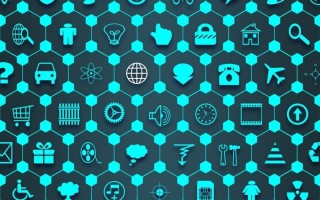
With the adoption of IoT, connected applications and systems are moving to the cloud. The number of end-devices and data generated on the cloud is also increasing.
With the adoption of IoT, connected applications and systems are moving to the cloud. The number of end-devices and data generated on the cloud is also increasing. Edge devices like sensors, mobile devices, wearables, robots, and many other connected devices in IoT ecosystem generate a huge amount of decentralized data.
Due to lack of reliable connectivity, delays and difficulties in processing this huge data on cloud, there is a challenge in analyzing and extracting important insights from this data. To deal with this challenge, enterprises are leveraging edge analytics along with cloud computing.
This combination brings stability in the IoT network by bringing the computational power near to the source of data and reducing the delays in analytics, resulting in real-time insights and resolutions for the problems of various industries. In other terms, when data cannot be taken to the algorithm, edge analytics brings algorithms to the data and provide important insights.
Analytics on edge
In recent years, due to advancement in semiconductor technology, MCUs and Processors are equipped with more processing power, specialized hardware components and computation capabilities which help with faster analytics on edge by deploying advanced machine learning methods such as deep neural networks or convolutional neural network.
Model developed on popular frameworks like TensorFlow, keras, and Caffe can be deployed after optimization to run on inference devices like Andriod and micro-controllers. Inference engines designed considering capabilities of MCUs, like TensorFlow-Lite, TensorFlow-micro, CMSIS-NN etc. are available which can execute quantized model on the edge for faster analytics.
Edge analytics benefit organizations where data insights are needed at the edge. Let us see how edge analytics is helping multiple industries across the globe.
Automotive
According to a report titled “Global Markets for Automotive Sensor Technologies,” the average number of sensors used in a car has been increased from 50-60 to 100+ and it is going to be 200+ in near future which will generate huge amounts of data. Continuous reliable cloud connectivity is another challenge for moving vehicles. For instance, in the case of self-driving cars, delay in sending data to the cloud, analyzing that data, and performing action after that can make huge difference in the success and failure of self-driving cars.
Edge analytics in automotive will help companies collect, analyze, and process data in real-time, allowing to take necessary actions immediately. Also, intelligent applications like collision avoidance, traffic routing, eyes-off-the-road detection systems, etc. can be designed through artificial intelligence and machine learning on edge. This ensures optimized asset usage, low maintenance and passenger safety.
Healthcare
IoT powered healthcare devices can collect patient’s data. Edge analytics can analyze the collected data without the need of continuous network connectivity. With advancement in semiconductor technology, the hardware and machine learning methods have become more efficient, thus more complex parameters can be monitored and analyzed by edge devices, like neurological activity, cardiac rhythms, blood pressure, etc.
Through edge computing, patient management, remote monitoring, in-patient care, and health information management all becomes quicker. To illustrate, a doctor’s mobile or tablet device is the edge between the patient (data source) and the cloud. A clinician treating a patient with a mobile or tablet will be able to enter patient data into the analytics platform at the edge where it is processed and displayed in near real-time. This helps to treat patients faster reducing their visit frequency. Also, it adds a secure layer of computing power between the cloud and the device, thus safeguarding the patient data.
Manufacturing
In manufacturing units or plants, there are multiple sensors involved in any production line on edge devices which continuously measure parameters like temperature, humidity, pressure, etc. of goods and equipment. Connecting these to cloud and analyzing the data will be time consuming. Edge computing can process this data for analysis and implement or suggest required changes in the processes. ML on edge also enables predictive monitoring, with machine learning algorithms forecasting equipment breakdowns before they occur and scheduling timely maintenance, which helps in extending their life, reducing downtime and overall savings in the maintenance cost.
Analytics on cloud
Having seen the advantages of edge analytics, it is important to understand that it does not replace cloud, but complements cloud computing with real-time analytics as it is close to data source. There are few processes that will continue to execute in cloud.
- Training of the machine learning algorithm: The development of a machine learning algorithm depends on large volumes of data, from which the learning process draws many entities, relationships, and clusters before training the model. This can be carried out on cloud along with training the model.
- Processing power & storage capacity: Unbounded scalability for storage and processing power, ease of deploying analytics make cloud analytics non replaceable. Historic data is stored on cloud that can be useful in future as cloud-based analytics works on a larger variety of data. For instance, it can add historical data to streaming data or analyze all the output from all the devices using edge analytics.
- Taking advantage of all edge devices of an application being connected to single cloud, cloud enables to perform super analytics on edge analytics. Cloud has the means to manage and turn that data into meaningful predictions and analysis.
How edge analytics complements cloud?
Real-time decision-making in IoT systems is still challenging due to factors like latency, bandwidth, power consumption, cost, form factors, and various other considerations. This can be overcome by adding artificial intelligence to the edge.
- Less utilization of data bandwidth/transfer: Shifting large amounts of data to the cloud for processing can consume high data bandwidth and produce a noticeable lag that may have a negative impact on time-critical applications. To avoid this delay and eliminate dependence on a data bandwidth, data processing on edge can be executed.
- Eliminating the need of continuous connectivity to cloud: In industries like oil, gas, or mining, where company employees work on remote sites far from populated areas, the connectivity is non-existent. In such scenarios, sensors on edge devices such as robots can capture data, analyze it and monitor operating parameters whether or not inside their normal range of values.
- Real-time performance with faster processing: Edge computing dramatically reduces the amount of data that has to be sent over the network, thereby reducing network congestion and speeding up operation. Instead of running processes in the cloud, Edge Computing runs processes on local places like a computer, IoT device or Edge Server. By bringing computation to a network edge, long-distance communication between a client and server is reduced and real-time insights are obtained.
- Enhanced data security (as closer to data source & location-aware): To explain, instead of having a security camera stream the content of its video feed up to the cloud to be analyzed for certain situations (unknown people, objects, etc.), that analysis can be done within the camera itself. Data privacy and security concerns associated with biometric data make it extremely important to only use the data locally on the device and not send it out over a cloud connection.
Cloud computing and edge computing are different approaches and purely depends on the application implemented. While they don’t discredit but complement each other. There can’t be one fit solution for all the scenarios. There are few key factors like real-time performance, cost of bandwidth, size of data, complexity of application, etc. which decides whether to go for edge analytics or cloud analytics or both (best of both worlds).
About the Author
Kaumil Desai is associated with VOLANSYS as a Delivery Manager past 3 years. He has vast experience in Product Development, Machine Learning on Edge, Complex algorithms design & development for various industries including Industrial Automation, Electrical Safety, Telecom, etc.