Computing-in-Memory Innovator Solves Speech Processing Challenges at the Edge Using Microchip’s Analog Embedded SuperFlash Technology
March 01, 2022
News
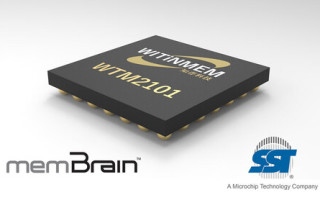
Microchip Technology via its Silicon Storage Technology (SST) subsidiary, announced that its SuperFlash memBrain neuromorphic memory solution is an embedded memory solution that simultaneously performs neural network computation and stores weights.
The company solved this problem for the WITINMEM neural processing SoC, the first in volume production that enables sub-mA systems to reduce speech noise and recognize hundreds of command words, in real time and after power-up.
Microchip has worked with WITINMEM to incorporate Microchip’s memBrain analog in-memory computing solution, based on SuperFlash technology, into WITINMEM’s ultra-low-power SoC. The SoC features computing-in-memory technology for neural networks processing including speech recognition, voice-print recognition, deep speech noise reduction, scene detection, and health status monitoring. WITINMEM, in turn, is working with multiple customers to bring products to market during 2022 based on this SoC.
“We are excited to have WITINMEM as our lead customer and applaud the company for entering the expanding AI edge processing market with a superior product using our technology,” said Mark Reiten, vice president of the license division at SST. “The WITINMEM SoC showcases the value of using memBrain technology to create a single-chip solution based on a computing-in-memory neural processor that eliminates the problems of traditional processors that use digital DSP and SRAM/DRAM-based approaches for storing and executing machine learning models.”
Microchip’s memBrain neuromorphic memory product is optimized to perform vector matrix multiplication (VMM) for neural networks. It enables processors used in battery-powered and deeply-embedded edge devices to deliver the highest possible AI inference performance per watt. This is accomplished by both storing the neural model weights as values in the memory array and using the memory array as the neural compute element. According to the company, the result is 10 to 20 times lower power consumption than alternative approaches along with lower overall processor Bill of Materials (BOM) costs because external DRAM and NOR are not required.
Permanently storing neural models inside the memBrain solution’s processing element also supports instant-on functionality for real-time neural network processing. WITINMEM has leveraged SuperFlash technology’s floating gate cells’ nonvolatility to power down its computing-in-memory macros during the idle state to further reduce leakage power in demanding IoT use cases.
For more information, visit the SST website