MLCommons Releases MLPerf Training v1.0 Results
July 01, 2021
News
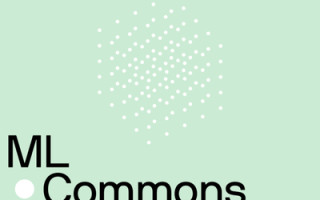
MLCommons released new results for MLPerf Training v1.0, the organization's machine learning training performance benchmark suite.
MLPerf Training measures the time it takes to train machine learning models to a standard quality target in a variety of tasks including image classification, object detection, NLP, recommendation, and reinforcement learning. In its fourth round, MLCommons added two new benchmarks to evaluate the performance of speech-to-text and 3D medical imaging tasks.
MLPerf Training is a system benchmark, testing machine learning models, software, and hardware. With MLPerf, MLCommons now has a way to track performance improvement over time. Per the company, compared to the last submission round, the best benchmark results improved by up to 2.1X, showing improvement in hardware, software, and system scale.
Similar to past MLPerf Training results, the submissions consist of two divisions: closed and open. Closed submissions use the same reference model to ensure a level playing field across systems, while participants in the open division are permitted to submit a variety of models. Submissions are additionally classified by availability within each division, including systems commercially available, in preview, and RDI.
As industry adoption and use cases for machine learning expands, MLPerf will continue to evolve its benchmark suites to evaluate new capabilities, tasks and performance metrics. With the MLPerf Training v1.0 round, the two new benchmarks for speech-to-text and 3D medical imaging, leverage the following reference models:
-
Speech-to-Text with RNN-T: RNN-T: Recurrent Neural Network Transducer is an automatic speech recognition (ASR) model that is trained on a subset of LibriSpeech. Given a sequence of speech input, it predicts the corresponding text. RNN-T is MLCommons’ reference model and commonly used in production for speech-to-text systems.
-
3D Medical Imaging with 3D U-Net: The 3D U-Net architecture is trained on the KiTS 19 dataset to find and segment cancerous cells in the kidneys. The model identifies whether each voxel within a CT scan belongs to a healthy tissue or a tumor, and is representative of many medical imaging tasks.
The latest benchmark round received submissions from 13 organizations and released over 650 peer-reviewed results for machine learning systems spanning from edge devices to data center servers. Submissions this round included software and hardware innovations from Dell, Fujitsu, Gigabyte, Google, Graphcore, Habana Labs, Inspur, Intel, Lenovo, Nettrix, NVIDIA, PCL & PKU, and Supermicro. To view the results, visit https://mlcommons.org/en/training-normal-10/.
Additional information about the Training v1.0 benchmarks is available at https://mlcommons.org/en/training-normal-10/.