MLPerf Results Show Increase in AI Performance
July 07, 2023
News
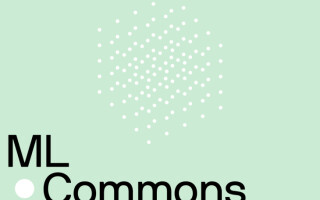
MLCommons announced new results from two industry-standard MLPerf™ benchmark suites: Training v3.0, which measures the performance of training machine learning models, and Tiny v1.1, which measures how quickly a trained neural network can process new data for low-power devices in small form factors.
The benchmarks show improvement in training advanced neural networks and AI model deployment at the edge.The latest MLPerf Training round also demonstrates wide industry participation and highlights performance gains of up to 1.54x and 33-49x over the first round.
The open-source and peer-reviewed MLPerf Training benchmark suite also features full system tests that emphasize machine learning models, software, and hardware for a broad range of applications.
In this round, MLPerf Training added two new benchmarks to the suite. The first is a large language model (LLM) using the GPT-3 reference model that reflects the adoption of generative AI. The second is an updated recommender, modified to be more representative of industry practices, using the DLRM-DCNv2 reference model. The new tests are designed to help advance AI by ensuring industry-standard benchmarks are representative of the latest trends in adoption and can help guide customers, vendors, and researchers alike.
Per the company, the MLPerf Training v3.0 round includes over 250 performance results, an increase of 62% over the last round, from 16 different submitters: ASUSTek, Azure, Dell, Fujitsu, GIGABYTE, H3C, IEI, Intel & Habana Labs, Krai, Lenovo, NVIDIA, NVIDIA + CoreWeave, Quanta Cloud Technology, Supermicro, and xFusion. In particular, MLCommons would like to congratulate first time MLPerf Training submitters CoreWeave, IEI, and Quanta Cloud Technology.
The MLPerf Tiny benchmark suite collects different inference use cases that involve "tiny" neural networks, typically 100 kB and below, that process sensor data, such as audio and vision, to provide endpoint intelligence for low-power devices in small form factors. MLPerf Tiny tests these capabilities, in addition to offering optional power measurement.
In this round, the Tiny ML v1.1 benchmarks include 10 submissions from academic, industry organizations, and national labs, producing 159 peer-reviewed results. Submitters include: Bosch, cTuning, fpgaConvNet, Kai Jiang, Krai, Nuvoton, Plumerai, Skymizer, STMicroelectronics, and Syntiant. This round includes 41 power measurements, as well. MLCommons congratulates Bosch, cTuning, fpgaConvNet, Kai Jiang, Krai, Nuvoton, and Skymizer on their first submissions to MLPerf Tiny.
“With so many new companies adopting the benchmark suite it’s really extended the range of hardware solutions and innovative software frameworks covered. The v1.1 release includes submissions ranging from tiny and inexpensive microcontrollers to larger FPGAs, showing a large variety of design choices,” said Dr. Csaba Kiraly, co-chair of the MLPerf Tiny Working Group. “And the combined effect of software and hardware performance improvements are 1000-fold in some areas compared to our initial reference benchmark results, which shows the pace that innovation is happening in the field.”
To view the results for MLPerf Training v3.0 and MLPerf Tiny v1.1, and to find additional information about the benchmarks please visit: Training v3.0 and Tiny v1.1.