Machines Watching Machines: The Value of AI-based Predictive Maintenance in Reducing Manufacturing Downtime
October 15, 2019
Blog
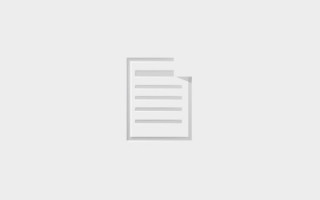
Manufacturers have always worked to minimize equipment failures resulting in unplanned downtime and have developed multiple techniques and processes along the way to mitigate the impact.
According to the World Bank, in 2017 (the latest year data are available) the worldwide manufacturing economy added $13.17T in value to the global GDP. Various sources estimate that anywhere from 4 percent to 20 percent of manufacturing capacity is lost to unplanned downtime (depending on the particular company and industry). Choosing a conservative 5 percent number averaged across all companies and industries means that the $13T number is 5 percent lower than it otherwise might be - an astonishing $693B in global productivity lost to unexpected maintenance issues for manufacturers. Reducing that number even slightly has huge potential benefits to the world's economy.
Of course, manufacturers have always worked to minimize equipment failures resulting in unplanned downtime and have developed multiple techniques and processes along the way to mitigate the impact. However, those techniques have generally been based on regularly scheduled maintenance (whether or not needed by a particular machine) and on humans collecting and comparing regular machine operational data manually. Both approaches have obvious and significant drawbacks.
By moving Internet of Things (IoT) data collection and analysis right to the industrial equipment itself and applying AI-based analytics, manufacturers have the potential to dramatically increase their predictive maintenance capabilities and correspondingly reduce their downtime costs. For example, within a factory, endpoint IoT nodes can regularly and continuously collect voltage, current, sound, temperature, motion and/or vibration data for each piece of equipment used in the manufacturing process. Then that data can be AI-analyzed in real time to search for patterns that have been known to precede equipment failures in the past.
This "machines watching machines" approach prevents unnecessary downtime for unneeded preventative maintenance as well as for unexpected real-time equipment failures. All of the equipment used in the manufacturing flow can be monitored all the time, very reliably, and taken offline only when needed to avoid imminent failure. No humans need to intervene except to manage equipment maintenance.
In the recent past, it was common for data to be AI-processed centrally, with the sensors themselves acting only as "dumb" data collection points. However, the combination of endpoint appropriate AI tools with increasingly capable endpoint IoT processing now allows "dumb sensors" to be transformed into "smart sensors" - enabling real-time data analysis at the endpoints. This transformation improves the value of the IoT network for manufacturers by avoiding potential latency, security and reliability issues. New toolkits and platforms make implementing this type of AI-based smart sensor network quick, easy and cost effective. ML frameworks simplify the ML programming tasks and so-called automated machine learning (or AutoML) tools further by selecting and optimizing AI algorithm hyperparameters using automated search methods that are essentially AI for building AI. In this way, these tools now allow developers without data-science expertise to be proficient when aided by such tools. Popular frameworks like TensorFlow, and Caffe ease the process for cloud-centric AI code building and tools like RapidMiner incorporate AutoML features as well.
While great for cloud-based AI processing, the demands of IoT sensor developers add yet another layer of complexity in that the target devices have far more constrained computing resources than essentially unlimited cloud computing offers. Enter the latest breed of AutoML tools such as the SensiML Analytics Toolkit that provide not only automation of AI code generation and hyperparameter tuning, but also intelligent selection of the underlying AI algorithm and input feature transformations with microcontroller targets in mind. Thus, while cloud AI automation tools can help developers optimize performance using complex deep learning algorithms and very large datasets, tools like SensiML allow developers to build high performing models where every last byte of RAM and clock cycle counts. Using a profiled library of feature transformers and a broad array of ML classifiers, these tools build practical sensor processing algorithms that can be deployed on the kinds of microcontrollers already being used simply to digitize physical sensors and relay raw data elsewhere in the network. Harnessing the full capabilities of modern 32-bit microcontrollers like ARM Cortex-M class SoCs, tools like SensiML can preprocess raw data from one or many physical sensors right at the endpoint and communicate a much lower volume of meaningful data for subsequent networked application use.
When applied to predictive maintenance, which often demands high rate vibration, ultrasonic, sound, and acoustic emission sensing - these IoT endpoint AI algorithms offer to greatly increase the current state-of-the-art for minimizing unplanned downtime. By ubiquitously applying well-tested methods previously practical only in very high-value applications, such methods can be implemented factory-wide. So, we expect smart endpoints to be the wave of the future when it comes to managing predictive maintenance for factories around the world - and we should all benefit from the increased productivity that wave will bring about.