AI & ML: Optimizing EVCS Location and Battery Management
May 30, 2023
Blog
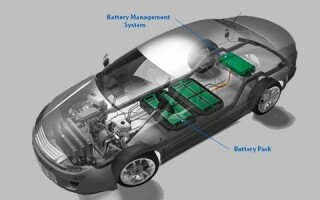
In a recent announcement, Google introduced a new feature designed for EV owners that leverages AI technology to identify the most suitable EV station and optimal route, providing users with enhanced convenience and efficiency. This development highlights the significant role AI can play in streamlining the charging experience for EV owners and further advancing the industry.
The electric vehicle (EV) market is experiencing rapid growth with a projected market volume of US$858.00 billion by 2027 and a remarkable compound annual growth rate (CAGR) of 17.02% from 2023 to 2027. As the demand for environmentally friendly transportation continues to surge, the integration of artificial intelligence (AI) and machine learning (ML) solutions in the EV sector holds immense potential to revolutionize the industry.
In this blog, we will delve into the transformative power of AI and ML technologies in enhancing the efficiency and effectiveness of electric vehicle charging stations (EVCS) and battery management. By harnessing the capabilities of AI and ML, we can unlock new possibilities for expanding the EV infrastructure and improving the performance and longevity of EV batteries.
Let us delve into the potential of AI and ML solutions in shaping the future of electric vehicles and creating a sustainable and efficient transportation ecosystem.
AI & ML for Optimal EVCS Location
EVCS are categorized into Level 1 (120 V) (240 V), Level 2, and DC fast charging based on charging voltage. Tesla has installed 1,971 DC fast charging stations globally for user convenience. Level 1 EVCS are placed in parking lots, while DC fast EVCS are placed near high-volume road access points. ELaadNL, a Dutch EV-infrastructure provider, optimally places charging spots and uses data analytics, including ML, for planning EV infrastructure.
The strategic placement of Electric Vehicle Charging Stations (EVCS) plays a pivotal role in alleviating user range anxiety and fostering widespread acceptance of electric vehicles (EVs). Several factors come into play when determining the optimal positioning of EVCS, including charging demand, existing infrastructure, economic considerations, and power-grid security.
Employing multiple objective optimization functions (MOOP) allows for the identification of the most suitable locations for EVCS. These MOOPs (Multi Objective Optimization Problem) encompass various objectives, such as cost minimization, maximizing net present value (NPV), or prioritizing placement in less populated regions.
By leveraging MOOP techniques, stakeholders can ensure that an EVCS is strategically positioned to meet the evolving needs of the electric vehicle market while addressing key considerations for users and the overall charging infrastructure.
To tackle multi-objective optimization problems (MOOPs), computational intelligence (CI) techniques such as swarm intelligence and evolutionary algorithms can be employed. Additionally, machine learning (ML) algorithms are utilized to process data and build models. ML clustering algorithms are instrumental in creating zones, while ML methods are employed to model electric vehicle (EV) user demand and traffic occupancy.
The process of determining the most suitable location for electric vehicle charging stations (EVCS) involves addressing the MOOP through the application of computational intelligence techniques like Particle Swarm Optimization (PSO) and Genetic Algorithms (GA).
Machine learning (ML) algorithms, including K-means clustering, Bayesian networks, and neural networks, offer an alternative approach for identifying the optimal EVCS site without the necessity of formulating a MOOP. These ML algorithms provide valuable insights into identifying the most suitable placement for EVCS based on several factors and can contribute to efficient decision-making processes.
Agent-based models (ABM) offer a more realistic approach to determine the placement and sizing of electric vehicle charging stations (EVCS), as they consider the diverse characteristics of different agents.
ABM has proven effective in simulating and analyzing the potential impact of EVCS placement and charging capacity on the adoption of electric vehicles (EVs) and charging usage over both short and lengthy periods. This enables the development of efficient strategies for deploying EVCS.
Improving accessibility to charging stations and implementing a smart grid system can revolutionize the EV industry. AI plays a crucial role in mitigating congestion issues at charging ports by effectively managing and evaluating the resources and locations of charging stations.
Optimizing Battery Performance and Longevity through AI & ML
Battery management systems (BMS) play a vital role in the functioning of electric vehicles (EVs) by ensuring the battery pack operates safely and efficiently. These systems analyze the voltage, current, and temperature of individual battery modules to accurately estimate and diagnose the battery's state-of-charge (SOC) and state-of-health (SOH). By monitoring these parameters, the BMS enables optimal performance and longevity of the battery pack, enhancing the overall efficiency and reliability of the EV.
Accurately estimating the state-of-charge (SOC) and state-of-health (SOH) of battery cells in electric vehicles (EVs) is a complex task due to the inherent inconsistencies in battery-cell performance and nonlinear battery characteristics. Traditional methods like equivalent circuit models (ECM) and electrochemical models (EM) have been used for SOC and SOH estimation, but they often come with computational expenses and reduced accuracy.
Fortunately, machine learning (ML) techniques offer a promising solution to enhance SOC and SOH estimations while maintaining low computational requirements. ML algorithms such as neural networks (NN) and support vector machines (SVM) have demonstrated their ability to improve the accuracy of SOC and SOH estimations.
By leveraging large datasets and training these ML models, EV battery management systems can benefit from more precise and efficient estimations, leading to enhanced performance and longevity of the battery pack.
Models based on recurrent neural networks (RNN), such as LSTM and RNN-GRU architectures, have proven valuable in assessing battery history and dynamic aging. These models can effectively capture long-term battery characteristics. Additionally, hybrid models that combine machine learning (ML) techniques with equivalent circuit models (ECM) or electrochemical models (EM) can yield accurate predictions.
Despite considerable progress, there are still challenges to address in battery degradation modeling. Pure ML models struggle to accurately represent battery aging mechanisms, while external operating conditions that influence battery degradation are diverse and intricate.
Most research in this domain primarily focuses on the cell-level perspective rather than considering the battery-pack-level dynamics. Overcoming these obstacles requires further exploration and development of models that can effectively incorporate these complex factors and accurately simulate battery degradation processes.
The Battery Management System (BMS) plays a crucial role in mitigating the risks of battery failure during electric vehicle (EV) operations. To enhance the detection of battery cell or module failures, researchers are exploring machine learning (ML) approaches.
These ML techniques aim to identify the location, time, and cause of such failures at an early stage. Battery cell faults can arise from operational abuse or mechanical damage, and the identification of mechanical faults often necessitates monitoring changes in vibration patterns. By leveraging ML algorithms, it becomes possible to analyze these signals and detect potential issues with battery cells, enabling proactive maintenance and minimizing the likelihood of battery failures during EV operation.
Through ML techniques like recurrent neural networks (RNNs) and hybrid models combining ML and electrochemical models (EM), we can accurately evaluate battery history, dynamic aging, and predict battery degradation. ML-based state estimation offers lower computational demands and improved accuracy, making it a promising approach for real-time EV applications.
The application of machine learning (ML) for state estimation shows enormous potential in enhancing battery management in electric vehicles (EVs) by offering lower computational requirements and improved accuracy.
Further research is necessary to tackle the current challenges faced in real-time EV applications. ML approaches also hold promise for early detection of battery cell or module failures. Yet, the identification of mechanical faults relies on monitoring changes in vibration patterns, which requires ongoing research efforts. By addressing these areas, advancements can be made in optimizing battery management and ensuring the reliable performance of EVs.
Conclusion
In conclusion, the integration of AI and ML in the EV industry is revolutionizing the way we approach battery management and EV charging infrastructure. Through AI and ML algorithms, we can now determine the optimal location for EV charging stations, effectively reducing range anxiety, and increasing the adoption of electric vehicles.
eInfochips has expertise in Autonomous Vehicles, EV Charging, V2X, Telematics, Infotainment, and Multimedia, and helps its global clients deliver a valuable experience with safety and efficiency. eInfochips provides services from EV charging hardware to AI/ML and data analytics.
References -
- Understanding the Role of BMS in Electric Vehicles (einfochips.com)
- https://www.einfochips.com/blog/ev-charging-solutions-trends-to-watch-in-2023-the-future-of-ev-charging-infrastructure/
- https://www.sciencedirect.com/science/article/pii/S2542435121003500#:~:text=Artificial%20intelligence%20%28AI%29%20algorithms%20and%20controllers%20can%20provide,significantly%20improve%20the%20consumer%20appeal%20of%20electric%20vehicles