Getting Started with Digital Twins
December 14, 2020
Story
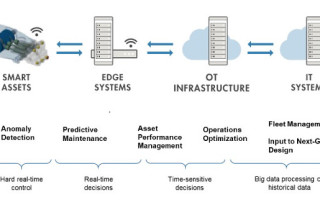
Simply put, today’s IIoT devices gather enough data to power virtual manufacturing equipment known as “digital twins” that businesses can use to automatically plan or test new strategies.
Industry 4.0 has been hyped-up for so long, that it’s easy to forget it’s a game-changer. Not because of the proliferation of industrial IoT devices that heralded its arrival, but what businesses could actually do with the data those devices generated.
Simply put, today’s IIoT devices gather enough data to power virtual manufacturing equipment known as “digital twins” that businesses can use to automatically plan or test new strategies. With digital twins, engineering and design teams can create autonomous systems capable of analyzing the current performance of physical manufacturing equipment, comparing it to past data, and adjusting its settings accordingly.
In the following Q&A with Embedded Computing Design’s Rich Nass, MathWorks Industry Manager Philipp Wallner explains the reasoning behind digital twins and the value they can deliver to enterprises that adopt – and, more importantly, know how to use them.
What are digital twins?
Philipp Wallner (PW): Digital twins are digital representations of a real-world physical asset in operation that reflects the asset’s current condition based on relevant, and easily accessed, historical data. Companies are using digital twins to analyze the performance of assets such as components, machinery, and entire production (or manufacturing) plants in operation and compare it to past data. They can also use them to optimize their company’s operations, predict future behavior, or ensure a given asset is running in top condition. For example, through the predictive maintenance of pumps, engines, power plants, manufacturing lines, or vehicle fleets.
How do digital twins fit within the larger context of Industry 4.0?
PW: A primary goal for manufacturers embracing Industry 4.0 should be transferring parts of the decision-making process that follow specific repeated patterns (that the AI algorithm can learn), while having new insights and ideas to come from human decision makers in the future. By doing this, engineers have more bandwidth to incorporate human insights (applying their domain expertise) into their company’s processes. The models powering these platforms use both historic data and the vast troves of new information generated by an asset’s sensors to respond appropriately no matter the situation. Digital twins add another layer to this process, giving engineers the opportunity to evaluate the model’s decisions, adjust variables, and test new scenarios without using assets in the physical world.
How do digital twins work?
PW: It depends on the company. For example, say you’re in the oil and gas industry and your company owns wells at three different locations, each with multiple pumps. You can use digital twins for predictive maintenance purposes, by having your engineers build digital twins for each pump and constantly updating the digital twins with data generated by sensors that evaluate each pump’s current operating condition.
Why should companies use digital twins?
PW: Engineers at any given company can use digital twin models in several ways to ensure their equipment is always running in peak condition:
- Equipment Downtime Reduction: They can use digital twins to improve the performance of components such as seals, valves, and plungers by predicting equipment failures and preventing them in advance.
- Inventory Management: Engineers can improve the company’s inventory management by using digital twins to identify which parts may need repair or replacement.
- Fleet Management, What-If Simulations, and Operational Planning: Referring back to the oil and gas use case I mentioned earlier is the easiest way to describe this one. So, each of the well’s pumps may have similar functionality; however, each is in a location with unique environmental factors that affect how they operate, such as temperature. Fortunately, the company can use digital twins to monitor the whole fleet of wells (or other infrastructure piece being monitored), identifying ways to increase efficiency and simulating future scenarios to test them, optimizing future operations.
Can you expand on a digital twin’s ability to capture a physical asset’s condition?
PW: Digital twins are frequently updated to match the physical asset’s current state, potentially allowing them to measure its condition over time. The information catalogued changes based on how the digital twin is being used and what it’s capturing. For example, digital twins in predictive maintenance use cases can be used for fault classification that will capture operational data from a pump’s healthy and faulty states, which engineers can compare to the digital twin histories of other pumps to understand how similar circumstances affected the pump’s behavior and the fleet's overall efficiency. More digital twins are needed to capture an asset’s holistic state.
Can those monitoring capabilities be used to detect anomalies as well?
PW: Digital twins run parallel to physical assets and flag operational behavior, which includes anomalies. When anomalies are detected, the digital twin can assess whether to flag them as potential equipment damage.
Can they be used for predictive maintenance?
PW: Because they can monitor entire fleets, digital twins are uniquely well suited to operational planning and asset maintenance. Companies running industrial automation and machinery applications, for example, can use digital twins to determine their equipment’s remaining lifespan and the best time to service or replace it.
It’s worth noting that since predictive maintenance generally requires making real-time or time-sensitive decisions, digital twins conducting predictive maintenance should be integrated directly on an industrial controller on the asset itself or at the edge, rather than on the on the IT/OT layers, as indicated below.
(Figure 1: A characteristic smart connected system topology and where digital twins should be deployed. © 1984–2020 The MathWorks, Inc.)
One benefit proponents of artificial intelligence like to focus on is its ability to help companies develop new strategies – would you say digital twins deliver that benefit to manufacturers?
PW: Absolutely. Digital twins can be used to simulate multiple scenarios in order to examine how factors such as fleet size, weather, or operating conditions affect a company’s performance. With the help of engineers, business owners can then use the information to make informed decisions about asset management, maintenance schedules, optimizing operations.
Can the factors that make digital twins effective at detecting anomalies be used to optimize operations?
PW: Of course. As mentioned before, companies can change variables such as fleet size, weather, performance, or energy costs and apply them to hundreds or thousands of scenarios to gauge their readiness or identify which adjustments are most likely to produce the best outcomes. This approach allows companies to mitigate risk, reduce costs, or maximize system efficiencies – sometimes all three.
For example, one of our machine builder customers in the food and beverage industry created a digital twin that optimized not only the design of the company’s robotic handling devices but also its fault testing and predictive maintenance capabilities. You can learn more about the example here.
How can readers build a digital twin?
PW: Engineers will be increasingly asked to develop digital twins for their company given the many benefits I’ve outlined so far. There are three primary models that design teams should keep in mind as they prepare, build and apply their digital twin: Data-driven, physics-based, and one that combines the two.
Can you walk us through them?
PW: Gladly. A company trying to optimize maintenance schedules by estimating an asset’s remaining useful life (RUL) should use a data-driven model. The company can also use similarity models if they have access to complete histories from similar assets, but if the company only has access to failure data, survival models can be used. If the company doesn’t have access to failure data but knows the asset’s safety threshold, degradation models can be used to estimate RUL, with the asset’s condition assessed by sensors measuring factors such as flow, pressure, and vibration in the case of a pump.
If a company wants to experiment with potential future scenarios and simulate how a fleet will behave under multiple conditions, it should use a physics-based model, created by connecting hydraulic and mechanical components. Physics-based models are fed an asset’s data, after which engineers are invited to adjust or add multiple variables or environment conditions, then view the simulated outcome.
Another digital twin option available to engineers is a Kalman filter, which combines physics and data by modeling an asset’s degradation, then periodically updating the digital asset’s state to represent the physical asset’s present condition.
How can readers incorporate digital twins into the design process?
PW: When designing an asset, engineering teams should create a digital twin for each component. The more digital twins they have corresponding to their asset’s components, the more accurate the digital twin’s measurements will be and the better equipped the asset’s engineers will be to virtually test their asset. Certain applications may require a lower total number of unique twins than others – teams modeling a system of multiple systems, for example, might not need a twin for each system because the expectations for precision may be lower. But if design teams intend to run, for example, failure prediction and fault classification, they need to create different models for each purpose, and the more digital twins available for those models, the better.
Which makes sense – the more data engineering teams have, the more value they can deliver to employers?
PW: Exactly. Digital twins’ flexibility and benefits should make them a top priority for businesses making the transition to Industry 4.0. By providing up-to-date representations of assets in operation companies give their engineering and design teams endless opportunities to optimize, automate, or improve the efficiency of their assets and evaluate future performance – all activities which lead to cost savings, accelerated development, and ultimately, industry leadership.
Philipp Wallner is Industry Manager for Industrial Automation & Machinery with MathWorks.