MLCommons Releases MLPerf Tiny Inference Benchmark
June 16, 2021
News
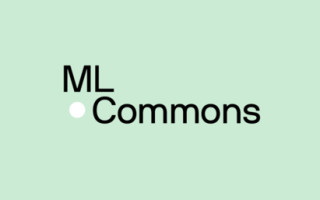
MLCommons launched a new benchmark, MLPerf Tiny Inference, to measure how a trained neural network can process new data for low-power devices in small form factors and included an optional power measurement.
MLPerf Tiny v0.5 is the organization's first inference benchmark suite that targets machine learning use cases on embedded devices.
The new MLPerf Tiny Inference benchmark suite is designed to capture a variety of use cases that involve "tiny" neural networks, typically 100 kB and below, that process sensor data such as audio and vision to provide endpoint intelligence.
The first v0.5 round included five submissions from academic, industry organizations, and national labs, producing 17 peer-reviewed results. Submissions this round included software and hardware innovations from Latent AI, Syntiant, PengCheng Labs, Columbia, UCSD, CERN, and Fermilab. To view the results, please visit https://www.mlcommons.org/en/inference-tiny-05/.
As a new benchmark, MLPerf Tiny Inference enables reporting and comparison of embedded ML devices, systems, and software. Developed in partnership with the Embedded Microprocessor Benchmark Consortium (EEMBC), the benchmark consists of four machine learning tasks that encompass the use of microphone and camera sensors with embedded devices:
- Keyword Spotting (KWS), which uses a neural network that detects keywords from a spectrogram;
- Visual Wake Words (VWW), a binary image classification task for determining the presence of a person in an image;
- Tiny Image Classification (IC), a small image classification benchmark with 10 classes; and
- Anomaly Detection (AD), which uses a neural network to identify abnormalities in machine operating sounds.
KWS has use cases in endpoint consumer devices, such as earbuds and virtual assistants. VWW has application use cases, for instance, with in-home security monitoring. IC has use cases for smart video recognition applications. AD has applications in industrial manufacturing for tasks such as predictive maintenance, asset tracking, and monitoring.
Per the company, with the addition of MLPerf Tiny, MLCommons covers the full range of machine learning inference benchmarks. These benchmarks range from cloud and datacenter benchmarks that consume kiloWatts of power down to IoT devices that consume only a few milliWatts of power.
The MLPerf Tiny v0.5 inference benchmarks were created thanks to the contributions and leadership of our working members over the last 18 months, including representatives from: Harvard University, EEMBC, CERN, Columbia, Digital Catapult, Fermilab, Google, Infineon, Latent AI, ON Semiconductor, Peng Cheng Laboratories, Qualcomm, Renesas, SambaNova Systems, Silicon Labs, STMicroelectronics, Synopsys, Syntiant, UCSD, and VoiceMed.
The MLPerf Tiny working group recently submitted a paper to the NeurIPS benchmarks and datasets track that provides in-depth information about the design and implementation of the benchmark suite (https://openreview.net/pdf?id=8RxxwAut1BI).
Additional information about the MLPerf Tiny Inference benchmarks is available at the github repository.
For more information, visit: http://mlcommons.org/