MCUs Combine with Optimized Toolset to Bring AI to the Edge
May 30, 2024
Sponsored Blog
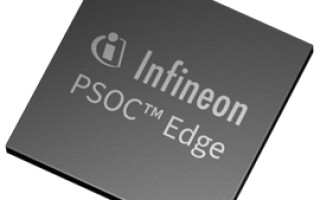
A growing number of designs in just about every application space are turning to AI for a plethora of reasons. As such, the technology is becoming an increasingly popular tool for the engineering community. The reasons for its popularity are many. For example, the end product can be more efficient by automating repetitive tasks, sifting through vast amounts of data for insights. By analyzing user behavior, market trends, and other relevant data points, designers can make more informed choices, leading to more effective designs.
This frees the design team to add creativity to the product, which can be inspired by AI. Today’s AI tools can explore new ideas, generate unique design elements, or experiment with unconventional approaches that they might not have considered otherwise.
And the cost factor is moving towards becoming a non-issue. The initial investment in AI tools may be higher, but they more than pay for themselves in the long run. That said, many silicon vendors are removing that potential impediment completely by offering open-source versions of the tools.
The Power of the MCU
The latest AI-enabled MCUs are providing enough compute power to handle many tasks at the Edge, and running the AI algorithms directly on devices rather than relying solely on cloud-based processing offers many advantages. For example, it would lower latency, increase privacy and security, reduce the need for off-prem communications, and result in a platform that’s easier to scale and customize.
The latency reduction comes from the shorter distances that data needs to travel between devices and sensors, a critical factor in industrial automation or IoT devices. And because the data never leaves the premises, no expensive communications medium is needed. This also leads to better control (and security) of the data.
Edge computing can distribute computational tasks across multiple devices, allowing for scalable and distributed AI deployments. This enables efficient resource utilization and accommodates changing demands, potentially on the fly. And implementing AI at the Edge allows for customization and optimization of algorithms tailored to specific device capabilities and constraints. This optimization helps improve performance and efficiency.
That said, not all of the available MCUs are suited for running Edge-based AI, due to a bunch of limitations, including limited processing power, memory constraints, and power efficiency (or inefficiency). MCUs typically have lower clock speeds and fewer processor cores compared to CPUs or specialized AI processors. This limits their ability to perform the heavy computations required for AI algorithms, especially those involving deep learning and neural networks.
AI models, particularly deep-learning models, often require substantial amounts of memory to store their results, and the amount of data involved can be huge. And while MCUs are generally designed for low-power operations, running AI algorithms, which involve intensive calculations, can significantly increase power consumption. This is a challenge for battery-powered or energy-sensitive Edge devices.
The PSOC™ Edge MCU
To address these limitations, semiconductor vendors like Infineon are developing more advanced MCUs and SoCs with built-in AI accelerators and improved capabilities for handling AI workloads. For example, the PSOC™ Edge is Infineon’s machine-learning-enhanced sensing, low power, secured, and advanced HMI high-performance microcontroller family that lets designers deliver unmatched highly usable end-user experiences for next-generation devices. In addition to the machine-learning hardware acceleration and the comprehensive peripheral set, PSOC™ Edge is supported by a rich set of design tools, including the popular ModusToolbox™ software and the Imagimob Studio AI solution.
Three devices currently make up the PSOC™ Edge series, all part of Infineon’s popular PSoC™ family of MCUs. They are designed with a multi-grained architecture offering a high-performance domain powered by Arm® Cortex®-M55 and Helium DSP support, as well as a low-power domain fueled by Cortex®-M33 core and Infineon’s NNLite neural net accelerator optimized for Always-On (AON) operation. This combination is well-suited for a host of consumer and industrial applications.
The PSOC™ Edge E81 is aimed at entry-level ML applications, and provides support for such Edge-device features as anomaly detection, predictive maintenance, acoustic event detection, keyword spotting, wake-word detection, voice prompts, and gesture/movement/presence detection. It also features an always-on acoustic activity-detection capability that enables HMI operation with low active and standby power consumption to support battery-powered products.
The PSOC™ Edge E83 adds an Arm® Ethos-U55 for advanced machine learning hardware offloading. It also features advanced ML capabilities providing voice/audio wake-word detection with always-on acoustic activity detection mechanisms for more complex AI/ML use cases such as natural language processing, beam forming, face recognition, and object detection. This combination enables friction-free HMI operation and low active and standby power consumption. Then there’s the PSOC™ Edge E84, which provides support for advanced graphics with a 2.5D GPU for rendering images and text and a display controller with MIPI-DSI/DBI interface with a resolution of up to 1024 by 768 pixels.
ModusToolbox™, an important element in the developer’s arsenal, is a comprehensive collection of development tools, software libraries, and embedded runtime assets boasting, among other capabilities, a feature-rich IDE that’s based on the Eclipse framework. Hence, it provides a familiar and robust platform for coding, debugging, and project management. Elements of the toolbox include BSPs, middleware, drivers, libraries, and sample applications. Tools for managing resources such as memory, power, and peripherals are integrated into the environment, helping optimize the performance and efficiency of the embedded application.
The second element, and a potential competitive advantage for Infineon, is its recent acquisition of Imagimob, a developer of AI and ML algorithms. The company specializes in developing production-ready AI models optimized for MCU-based Edge devices. The algorithms can be applied across various domains such as industrial automation, healthcare, consumer electronics, and automotive. In addition, Imagimob provides user-friendly development tools that simplify the process of creating, training, and deploying AI models on Edge devices. And as you would expect, the tools and software are optimized for the PSOC™ Edge MCUs.
Infineon has a long and deep history in this space, and the manufacturing expertise to handle all your “AI at the Edge” needs. It also has a large library of complimentary components that should cover most of your design. Contact the company online or send an email to [email protected] for more information.