How Machine Learning and Big Data Analytics Uncover Actionable Chip Design Insights
June 29, 2022
Story
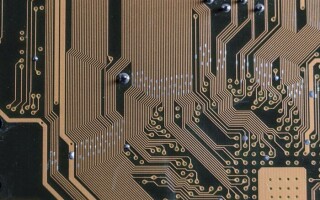
With increasing SoC and system complexity, shrinking market windows, and growing engineering resource challenges, anything that can improve chip design productivity—not to mention the associated chip quality—can give you a significant edge over your competitors.
It turns out that the raw ingredients needed to improve the design process have always been there for the taking—they lay in the interconnected electronic design automation (EDA) metrics and design-flow data being constantly generated by the tools. It has just been highly challenging to efficiently parse the massive volumes of data to find the actionable insights that can truly make an impact.
Cue the timely confluence of big data, data science, and data analytics technologies; through their unique coalescence, the vast, rich, and yet long untapped sources of information can be harvested, curated, and, most importantly, efficiently put to work to help accelerate the design process.
In this blog post, I’ll share how this new boost to the SoC design paradigm delivers measurable improvements in design-process visibility and observability, provides deep design analytics for a better, faster, data-driven way to design, and enables a knowledge-led culture across the entire business for smarter end-to-end decision-making.
Moving Beyond Spreadsheets and Connecting the Dots
An RTL-to-signoff design flow consists of numerous engines designed to co-enable the achievement of your targeted power, performance, and area (PPA) goals as smoothly and efficiently as possible. From this flow comes a high volume of primarily unstructured data. But suppose you can connect all the data points in that data in the right way. In that case, you can, for example, potentially uncover patterns that lead you to additional opportunities for design improvement or areas that you can address earlier to facilitate faster design closure.
Engineers try to find insights via log files, but this can get unwieldy, inexact, and difficult to share. It’s a time-consuming and manual process to extract data, place it into spreadsheets, and format it so that patterns and trends become apparent—and easily sharable. Yet, this is what engineers must do when creating project progress reports. What’s needed is a way to “connect the dots” via a more comprehensive, holistic view of the data. For example, it would be helpful to combine data associated with a timing issue in one part of the design with data about a congestion issue in another area…and see what is revealed.
Data visualization and design optimization technology help fill the void. For example, advanced data visualization can facilitate better decision-making by providing a unified, 360-degree of all tool flow data across all implementation projects and activities. From causation analysis to prescriptive solutions, targeted actionable insights can help unlock untapped PPA. And with easily sharable designer knowledge, teams can improve productivity and achieve faster design closure.
Smarter Data Use for Smarter Design
The Synopsys DesignDash product is an example of a data visualization and machine-intelligence- (MI-) guided design-optimization solution that can enhance the design process, foster more efficient designer workflows, and mitigate project risks. The solution autonomously siphons metrics data while also intelligently curating the associated analysis data directly from the unique single data model shared by tools in the digital design flow. The data is loaded into always-on, industry-standard databases, making the information easy to search, filter, graph, compare, share, and cross-reference via a web-based UI.
With machine learning capabilities, DesignDash technology reveals why something is happening. It autonomously classifies design trends, identifies design limitations, and provides guided root-cause analysis across the entire design flow. Whether a team needs to know about the primary cause of a design failure, how a critical path evolved structurally through each stage of a flow, or how a particular run compared to previous experiments, these questions and more can be answered with the technology.
Systemic and scale complexity is growing, while time-to-market pressures continue to become more aggressive. Getting a wide-ranging view of the underlying data for every design and project can complement the engineering ingenuity that drives achievements in chip design quality and design productivity. Data visualization, enhanced with machine learning capabilities, can lead to faster, smarter, and better design.
Mark Richards is a sr. staff product marketing manager at Synopsys. Before joining Synopsys, Mark held a variety of design and product engineering roles for companies including LSI Logic and 3Dlabs. He has a master’s of electronic and electrical engineering degree from the University of Birmingham.