Edge Visualization Can Be Complex but Carries Huge Benefits
March 03, 2022
Blog
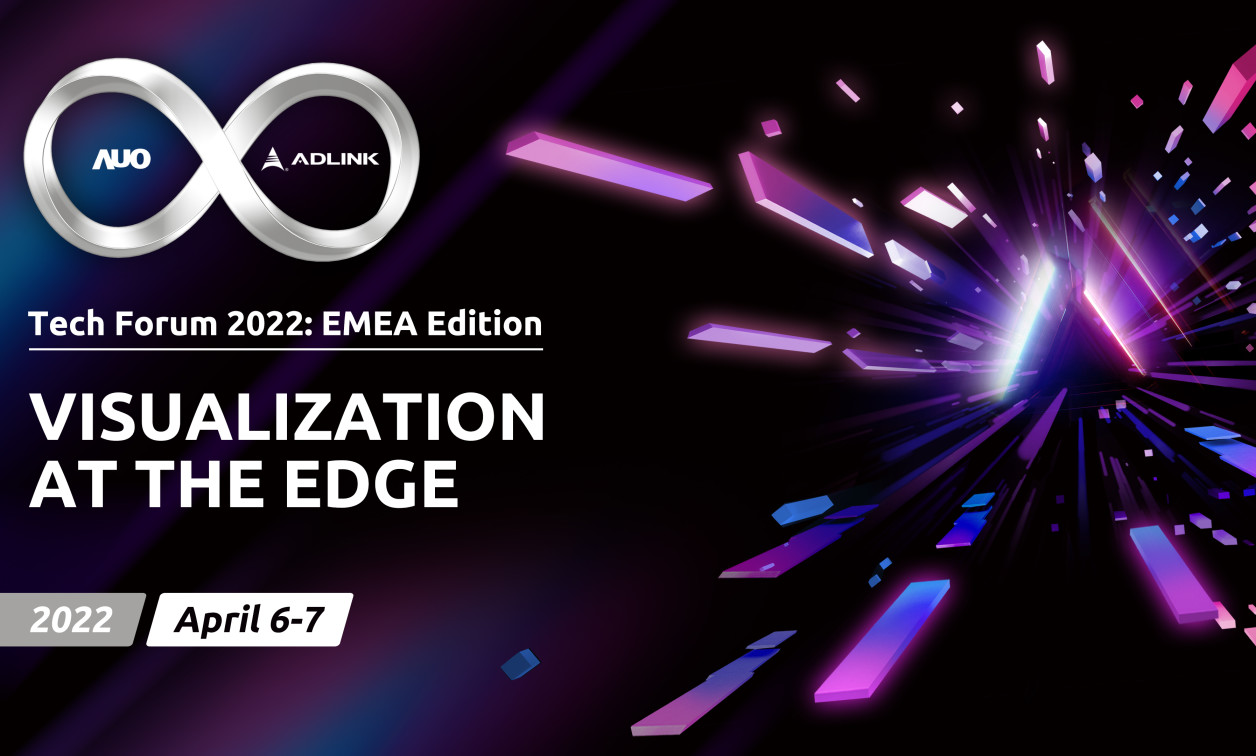
Edge visualization is one of those terms that if you ask 10 people for a definition, you’re likely to get ten different responses. To avoid that ambiguity, I went right to one of the sources of this technology, namely Jim Liu, the CEO of ADLINK Technology. As you might expect, it was not a simple answer.
Liu said, “The Edge side is about the operation, as it’s is where the data originates, and where decisions are carried out. But because visualization is involved, the amount of data that’s typically captured can be enormous. Hence the need for vast compute capability.”
Liu’s definition brought in yet another term, AI at the Edge or Edge AI, where the computing “follows” the data. That represents the initial actions that occur in the compute system after receiving the data.
In most cases, this technology is dependent on the microprocessors within the platform. And thanks to the latest innovations from the likes of Intel and NVIDIA, those capabilities are readily available. However, the complexity to design these platforms grows accordingly, particularly because power consumption can be a concern at the Edge.
Liu notes that many applications require high-end graphics to be displayed at some point, like in surgical system or other healthcare equipment. So it requires a combination of lots of compute power and the right panel. For that reason, ADLINK has partnered with AUO, one of the world’s leading panel manufacturers.
It’s no secret that AUO is looking to move from being a pure commodity panel vendor to something that’s more of a vertical visualization supplier, offering high-end features that allow it to compete on things other than price. And partnerships with the likes of ADLINK can make that happen far more quickly than trying to go it alone.
Computing at the Edge Vs. the Cloud
There’s a long list of both pros and cons when keeping data at the Edge versus the Cloud. On the positive side, the most obvious one is that it lets the system react far more quickly. The time that it takes for the data to travel from the Edge to the Cloud and back is eliminated. While that time can often be counted in milliseconds, those milliseconds add up and if I’m laying on an operating table or standing in front of a moving vehicle, I don’t want to wait those milliseconds, as they really could make the difference between life and death.
There’s also a cost savings associated with Edge-based computations as you are eliminating a potentially expensive transmission medium (5G cellular) to transmit your data. Then there’s the security aspect. If the data never leaves your facility, it’s less likely to get stolen.
On the other side of the coin, you usually already have lots of compute power in the Cloud. So you can reduce the cost of your compute engine at the Edge if you can live with the “pros” raised above.
A good example of an application that takes advantage of both Edge and Cloud AI is the automobile. Some decisions, like when to brake in an autonomous vehicle, are better made locally. But decisions regarding traffic routing, maintenance, etc., are likely better off handled in the Cloud because they are less time sensitive.
Healthcare is also a great application that can take advantage of Edge visualization while also making great use of the Cloud. As discussed, real-time responses are often required, but data storage in the medical space is essential, and the amount of data that’s collected is huge, especially when you consider the storage space needed to store a single image. Multiply that by the number of images per person, and the number of people that have any type of image recorded (X-rays, MRIs, etc.), and you end up with a very big number, one that can’t possibly be captured at the Edge. And don’t forget, those images are sometimes annotated, possibly with data-intensive voice, and stored in multiple locations, further increasing the memory footprint required, bringing it to obvious Cloud proportions.
It goes (almost) without saying that Edge visualization will have a big impact in a factory setting. Using machine learning, the factory can handle the timing for its own maintenance and minimize down time by flagging small issues before they become big issues. It can also help improve product quality on the fly.
In the aforementioned vertical applications, advanced or Edge-integrated displays play an important role in visualizing data and images for fast response and decisions. That role has increased even more, due to the COVID-19 pandemic, which has sped up digital transformation for global industries. Users are increasingly more dependent on human-machine interfaces (HMIs) for remote and contactless communications. The end result is a need for high-end displays, which is the specialty of AUO. The company offers leading-edge display technologies that include micro LED, mini LED, A.R.T. (Advanced Reflectionless Technology), and the latest in sensing technology.
To learn far more about Edge visualization, I suggest you check out the Tech Forum put on by ADLINK and AUO that takes place on April 6 and 7.