Storage
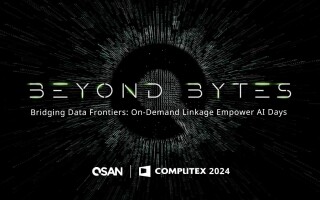
QSAN to Bring AI Storage to COMPUTEX 2024
April 25, 2024
Taiwan. At COMPUTEX 2024, QSAN will demonstrate its innovative solutions to advance enterprise in the future of AI. First, the XCubeFAS 5226 delivers unparalleled performance. It supports low latency, high throughput, and various IOPs to accelerate challenging workloads for AI applications.
Software & OS
Multimedia
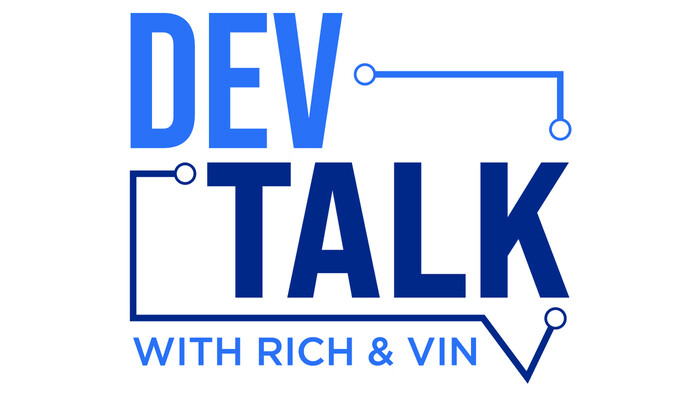
DevTalk with Rich and Vin (and Digi CEO)
April 25, 2024
Welcome to a slightly different version of our DevTalk with Rich and Vin podcast. In addition to doing our traditional tech talks, which you’ve clearly told us that you enjoy, we will be producing discussions with CEOs. But we won’t be going through the usual company blah, blah, blah. We’re going to dig a little deeper and find out what really drives these individuals, who are obviously very determined, or they wouldn’t be in the positions they are in. And we told them that to be a guest, they couldn’t limit or filter our questions in any way; anything and everything is on the table.
To get us started, we spent some time with Ron Konezny, the CEO of Digi International.
Analog & Power
-
Sustainable Irrigation through Embedded in India
April 19, 2024
-
Why You Should Consider .8mm PCBs Versus 1.6mm
April 12, 2024
-
e-peas Showcases Complete Solutions for Batteryless Design with Industry-Leading Ecosystem Partners at embedded world
April 05, 2024
-
embedded world 2024 Best in Show Nominees
April 01, 2024
AI & Machine Learning
-
Arm Pushes Edge AI to a Higher Level, Marries Embedded with AI and IoT
April 22, 2024
-
DEEPX has AI Acceleration at COMPUTEX 2024
April 19, 2024
-
Arm CEO Rene Haas to Deliver COMPUTEX Keynote
April 19, 2024
-
COMPUTEX 2024: Unlocking Innovative AI
April 18, 2024
Automotive
-
Application Highlight: ADLINK ADM-TJ30 Brings AI to ADAS
April 08, 2024
-
NXP Releases its Open S32 CoreRide Platform Simplifying SDV Development
March 28, 2024
-
Cadence Expands Tensilica Vision Family with Radar Accelerator and New DSPs for Automotive Applications
March 11, 2024
-
Ethernovia Unveils Single and Quad Port, 10G to 1G Automotive PHY in 7nm
March 05, 2024
Debug & Test
-
Rohde & Schwarz Presents Test Solutions for Embedded Systems
April 22, 2024
-
Embedded Testing Vs Software Testing – Key Differences
April 15, 2024
-
Debug and Analyze with PLS Development Tools During ew '24
February 05, 2024
-
TASKING Supports Arm Architecture for Automotive Development
January 29, 2024
IoT
-
New Book on Rugged Edge Offers Insights on Industrial Computing
April 24, 2024
-
7L600: EKF Introduced its First ModBlox7-Based Product
April 22, 2024
-
Digi International's Digi Connect Sensor XRT-M Powered by Digi Axess
April 16, 2024
-
Gateways & Nodes for Raspberry Pi, Pico, Espressif, and More
April 15, 2024
Storage
-
Micron Releases a Global First
April 22, 2024
-
Embedded World 2024: High-Endurance, Robust Cross-Temp Reliability 176-Layer Storage, DDR5-5600 Solutions Take Center Stage at ATP Electronics’ Exhibit
April 05, 2024
-
New Greenliant eMMC and NVMe BGA SSDs on Display at embedded world 2024
April 05, 2024
-
embedded world 2024 Best in Show Nominees: Memory & Storage
April 01, 2024
Networking & 5G
-
Alif Semiconductor Announces BLE and Matter Wireless Microcontroller With Neural Co-Processor for AI/ML Workloads
April 10, 2024
-
Doubling Down on 5G: Integration of Two 5G Modules in One Device
April 10, 2024
-
How Single Pair Ethernet Streamlines Industrial Networks
April 04, 2024
-
Walter, an Open Source WiFi, BLE, LTE-M, NB-IoT, and GNSS Module for Prototyping and Production
April 02, 2024
Open Source
-
Semidynamics Drops its All-In-One AI IP On Us
April 11, 2024
-
DigiKey Hosts Great Board Giveaway, Technical Demos and More at Embedded World 2024
April 05, 2024
-
Circuit Playground Express: For Education, Pros Too?
April 03, 2024
-
Executive Viewpoint: Jason Kridner, BeagleBoard.org
April 02, 2024